Projects
Sunt in officia anim aute occaecat sed dolor sit nulla pariatur dolor duis velit veniam. Lorem ipsum cupidatat fugiat adipisicing dolore elit duis reprehenderit anim incididunt in incididunt deserunt sunt culpa fugiat eu minim veniam. Veniam laborum mollit commodo labore excepteur ut sunt dolore dolor in minim enim officia sit occaecat in cillum ullamco. Lorem ipsum ad tempor ea elit anim aliqua nulla exercitation veniam id non elit in in deserunt magna.
Learn more about each project at RENCI below.
A Climate-Forward Coastal Water Level Model Analysis for Coastal Inundation and Flood Risk Planning (MAPP)
With losses mounting from coastal flooding and associated impacts, better information on present and future coastal flood risk is needed by stakeholders ranging from the military to state and local governments who collectively oversee trillions of dollars of coastal infrastructure; small to large businesses in coastal economies; and roughly half of the US population who live in coastal areas. Available flood risk information is largely limited to present day 1% and 0.2% annual chance flood levels from the FEMA National Flood Insurance Program, does not take into account ongoing Sea Level Rise (SLR), and is insufficient for future planning. This project will extend the companion project "Reanalysis" to compute future climate-model based coastal hazards datasets using the ADCIRC storm surge, tide, and wind-wave model. Climate model projections are being analyzed to develop "transfer functions" that can be applied to the current climate conditions and then used to project coastal hazards data into future climates.
A Multi-decadal Coastal Water Level Model Reanalysis for Coastal Inundation and Flood Risk Assessment (Reanalysis)
The Renaissance Computing Institute (RENCI) at The University of North Carolina at Chapel Hill is conducting a series of long-term simulations of coastal water levels in the US east and Gulf of Mexico coastal regions. The ADCIRC storm surge, tide, and wind-wave model is used to compute the storm surge and tide response to coastal water levels for the period 1979-2022, forced by the European Centre for Medium-range Weather Forecasting (ECMWF) 41-year meteorological reanalysis known as ERA5. The resulting 44-year coastal water level dataset can be used for a variety of applications, including extreme water level characterization and boundary conditions for smaller, regional model applications.
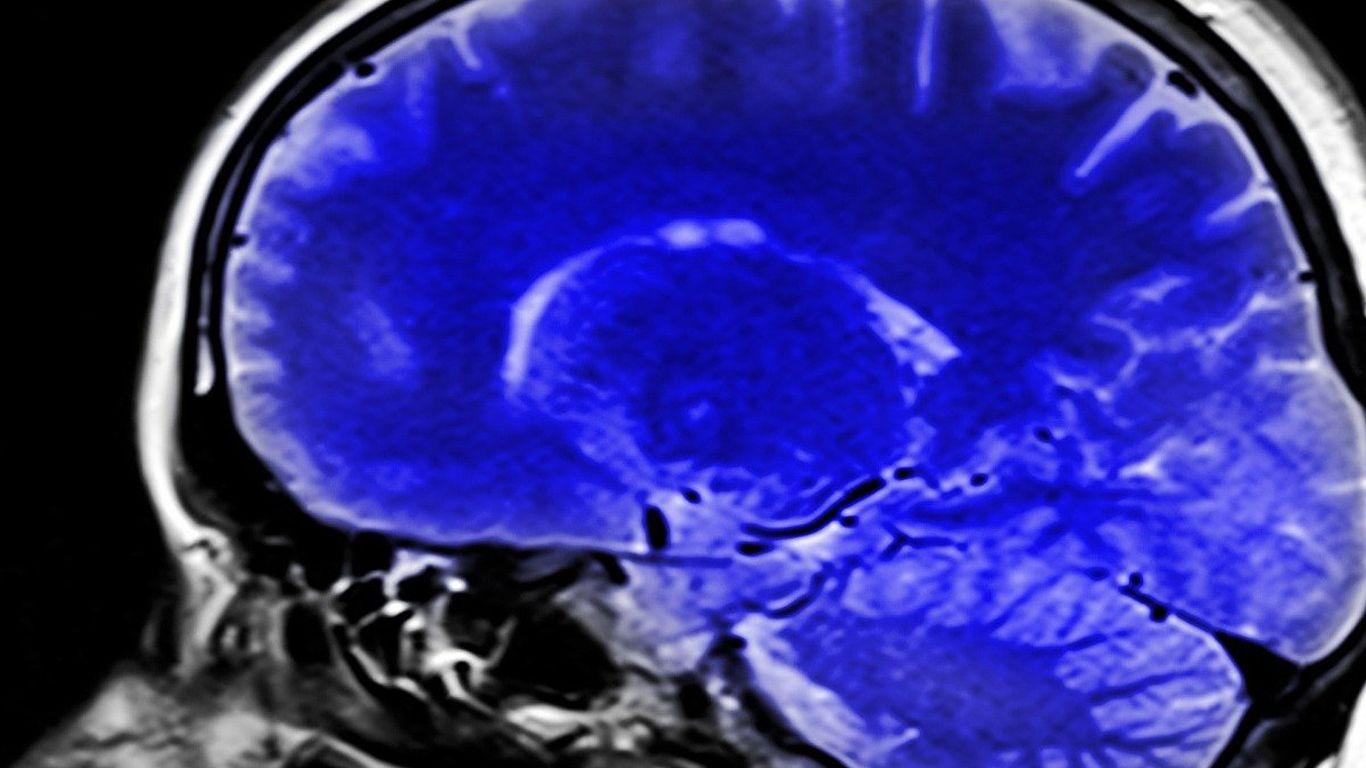
A Scalable Platform for Exploring and Analyzing Whole Brain Tissue Cleared Images
The ability to accurately localize and characterize cells in light sheet fluorescence microscopy (LSFM) images is indispensable for shedding new light on the understanding of three dimensional structures of the whole brain. We are designing a semi-automatic annotation workflow to largely reduce human intervention, and thus improve both the accuracy and the replicability of annotation across different users. The annotation software will be expanded into a crowd-sourcing platform which allows us to obtain a massive number of manual annotations in a short time. We will also develop a fully 3D cell segmentation engine using 3D convolutional neural networks trained with the 3D annotated samples. We will then develop a transfer learning framework to make our 3D cell segmentation engine general enough for the application of novel LSFM data which might have a significant gap of image appearance due to different imaging setup or clearing/staining protocol. This general framework will allow us to rapidly develop a specific cell segmentation solution for the new LSFM data with very few or even no manual annotations, by transferring the well-trained segmentation engine that has been trained with a sufficient number of labeled samples. Finally, we will apply our computational tool to several pilot studies including Autism mouse brain and human fetal tissue data.
Adcirc Viz: A Visualization Application for Distributed ADCIRC-based Coastal Storm Surge, Inundation, and Wave Modeling
ADCIRC Viz is a graphical user interface providing access to forecast outputs from the coastal storm surge, inundation, and wave modeling in the ADCIRC Surge Guidance System (ASGS). Its real-time prediction output improves decision makers’ ability to analyze, display, and communicate key information about the threats posed by hurricanes.
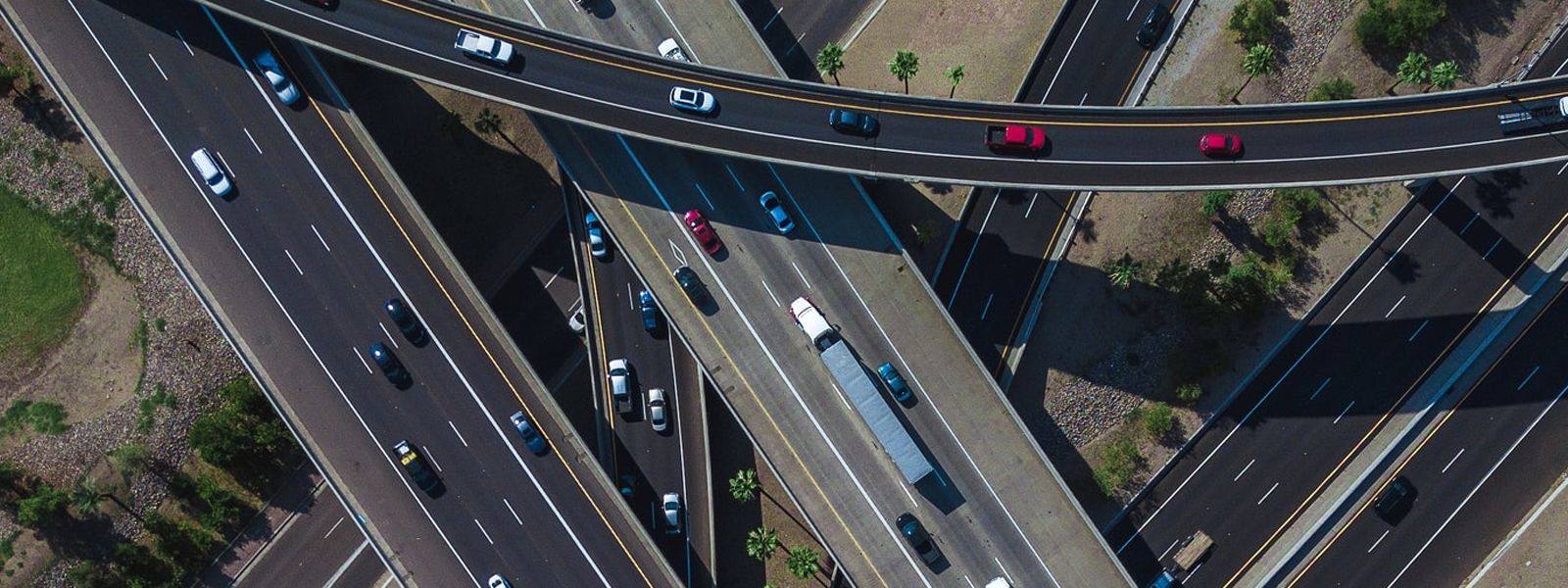
AERPAW
Aerial Experimentation Research Platform for Advanced Wireless AERPAW is a $24 million grant awarded by the National Science Foundation to develop an advanced wireless research platform, led by North Carolina State University, in partnership with Wireless Research Center of North Carolina, Mississippi State University and Renaissance Computing Institute (RENCI) at the University of North Carolina at Chapel Hill; additional partners include Town of Cary, City of Raleigh, North Carolina Department of Transportation, Purdue University, University of South Carolina, and many other academic, industry and municipal partners. AERPAW, the nation’s first aerial wireless experimentation platform spanning 5G technologies and beyond, will enable cutting-edge research — with the potential to create transformative wireless advances for aerial systems.
AIxB: Building a collaborative ecosystem for innovation in Artificial Intelligence and the Biological Sciences
A winner of the UNC Vice Chancellor for Research’s Creativity Hubs Initiative, AIxB takes a fresh look at AI technologies through the lens of biology to develop more inspired, interpretable and innovative AI while advancing biological science in a virtuous cycle of discovery. AIxB creates a collision space for convergent science, bringing together advances in knowledge graphs and explainable AI and biology. Together, the team will explore how the combinatorial histone code controls gene expression and thus cell functions to result in the rich biodiversity we can see every day, all without changing DNA sequence. This research has the potential to interpret the complexity of the histone code, in effect reducing experimental time and eventually informing therapies for treatable DNA-based diseases.
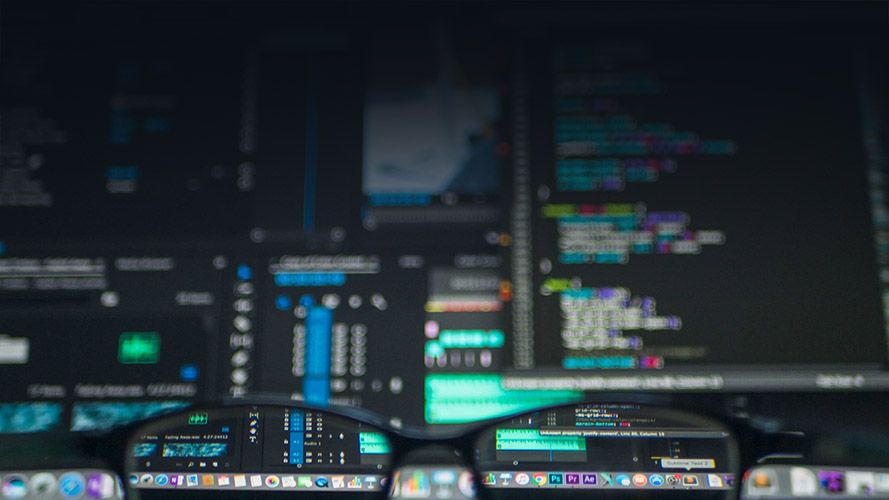
An Intelligent Concept Agent for Assisting with the Application of Metadata (INCA)
The goal of INCA (An Intelligent Concept Agent for Assisting with the Application of Metadata) is to develop an intelligent concept assistant that will allow researchers to generate and share sets of metadata elements relevant to their project and will use machine learning techniques to automatically apply this to data. The agent is based around a personalized dashboard of metadata elements that can be collected from multiple specialized portals, as well as sites such as Wikipedia. These elements can be coupled with classifiers that can be used to self-identify datasets to which they may be relevant, making the selection of appropriate vocabularies easier for researchers.
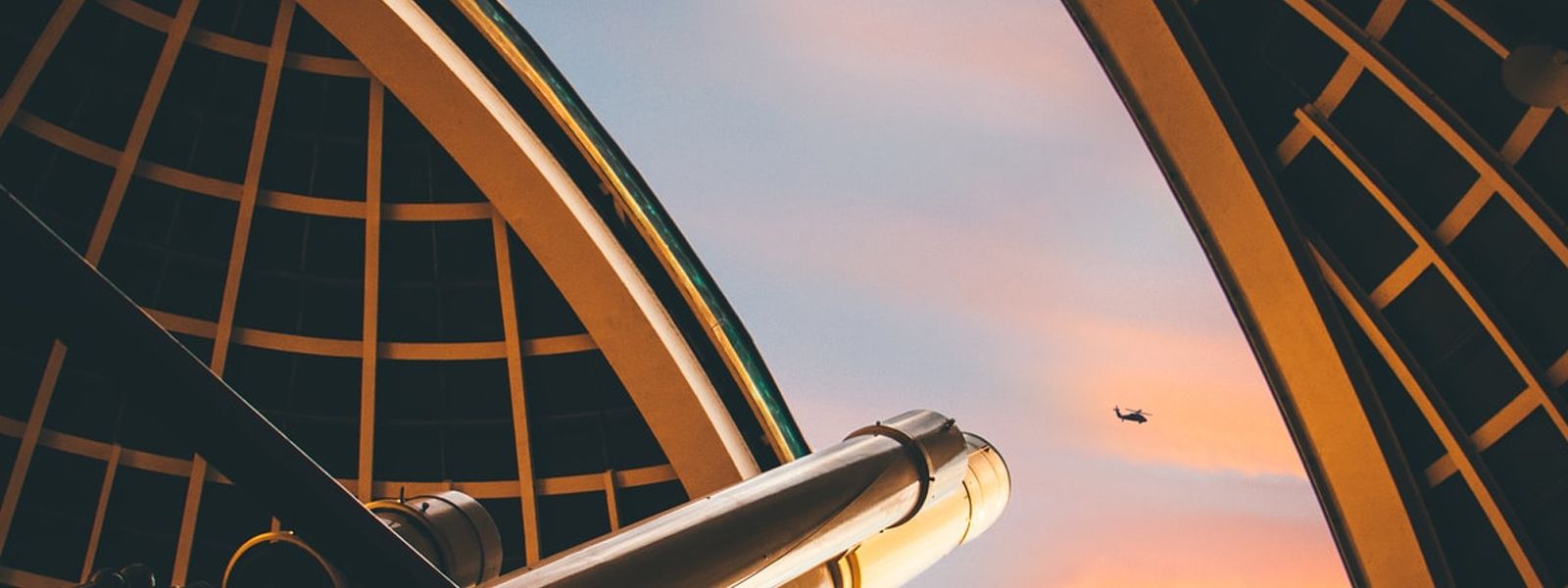
AtlanticWave-SDX
The AtlanticWave-SDX (AW-SDX) project aims to enable a new way for domain scientists and network engineers to use Open Exchange Points (OXP) to support science workflows via provisioning flexible inter-domain services through a single user- friendly interface. To achieve such a goal, the AMPATH OXP in Miami, SOX OXP in Atlanta, AndesLight OXP in Santiago, Chile, and SouthernLight OXP in Sao Paulo, Brazil were evolved to become Software-Defined Exchange (SDX) points. The AtlanticWave-SDX controller software is developed to link a large number of data sources and scientific users to the advanced distributed OXPs. Upgrade of the AW-SDX network infrastructure to support 100+ Gbps and further development of the SDX controller are essential to evolve the OXPs in Miami, Atlanta, and Latin America, (and also S. Africa) towards an open, innovative platform while continuously enhancing their production capacity and service capabilities. This project targeted to support an array of large-scale international science drivers: the Vera Rubin Observatory (a large optical telescope under construction in northern Chile), High Luminosity LHC Challenge and HEPiX, and Association of Universities for Research in Astronomy (AURA), etc.
BDC: Data Management Core
This project will (1) support data generators’ unique needs with training, consulting, and tools; (2) elicit consensus expectations from the community and communicate them widely; and (3) prioritize high-value data for ingest into the BioData Catalyst ecosystem. To develop, diversify, and sustain a scientific workforce capable of accomplishing the NHLBI’s mission, the team will make BDC’s data portfolio more appealing to a wider array of researchers, expanding and diversifying the community conducting secondary analysis on NHLBI data. RENCI’s role is to lead a landscape analysis of available tools, templates, and technologies to support ingestion of data into the BDC system.
BDC3: COVID-19 Data and Infrastructure Research
This project aims to fund the BioData Catalyst efforts focused on ingesting and making COVID-19 data sets available for research throughout the ecosystem, as well as to provide NIH with a broad assessment of COVID-19 and PASC data, infrastructure, and interoperability.
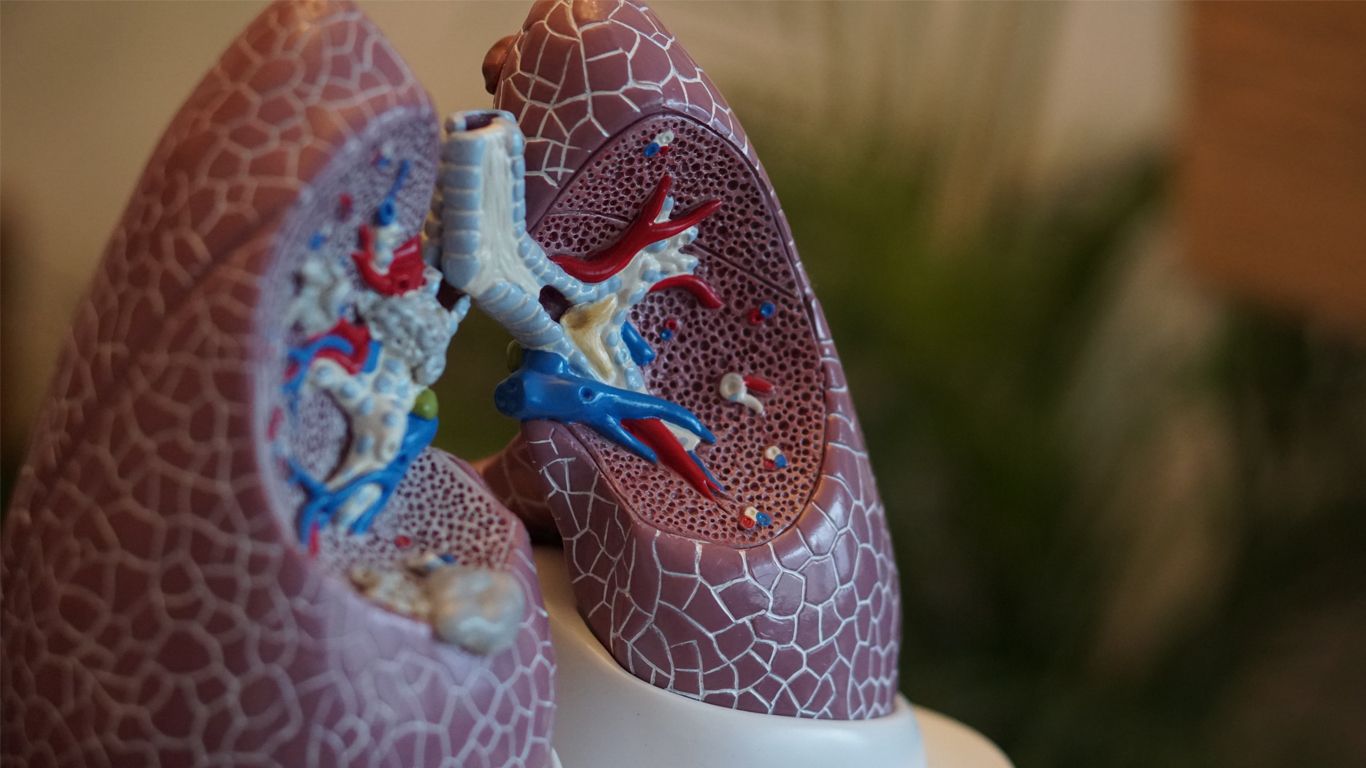
BDC3: Fellows Program
The NHLBI BioData Catalyst Fellows Program provides early-career researchers the opportunity to receive funding to support research on novel and innovative data science and data-focused research problems. The program is open to academic disciplines conducting biomedical research, particularly in heart, lung, blood, and sleep domains.
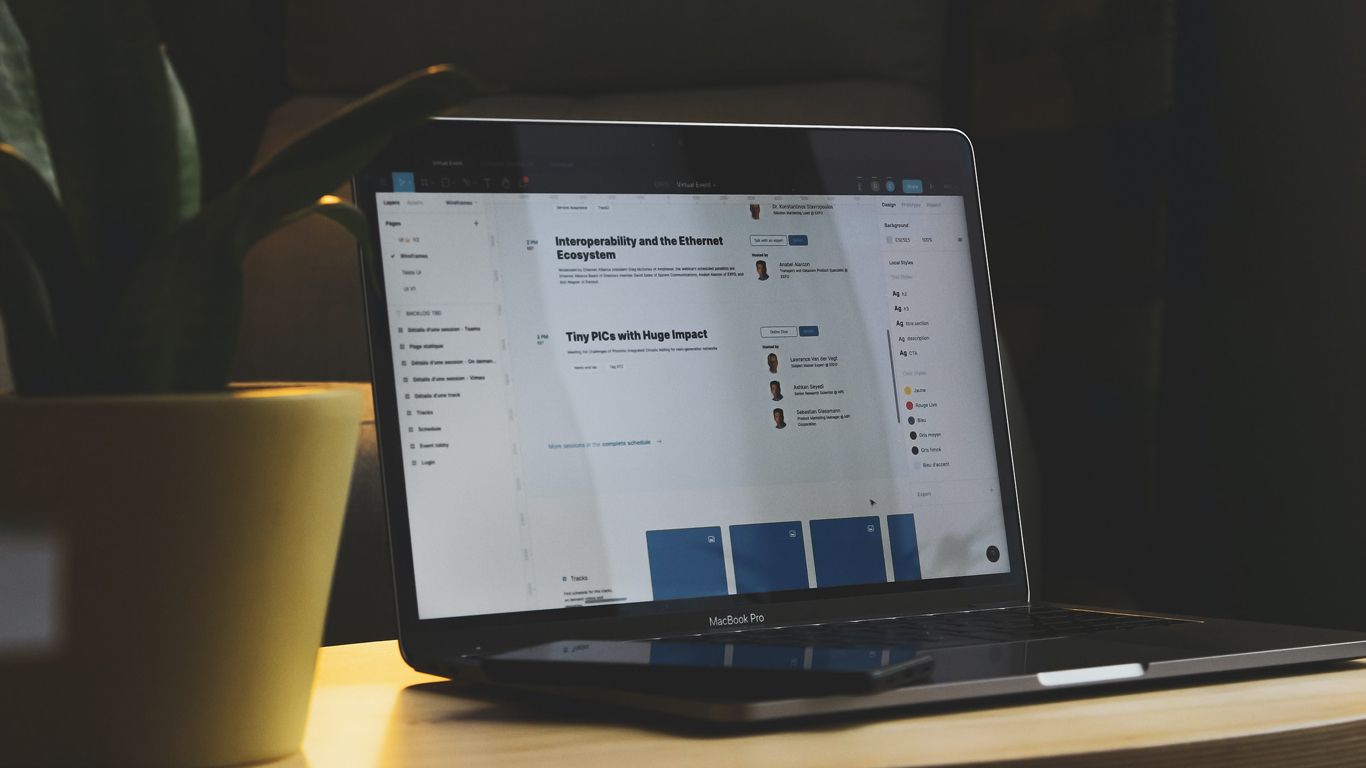
BDC3: The Cloud IC Interoperability Framework (CICI)
The NIH Cloud Institutes and Centers Interoperability (CICI) project is working to enable a cloud data and computing infrastructure demonstrating interoperability between NIH Institutes and Centers (ICs). CICI will leverage existing infrastructure to create a cloud-native semantic fabric enabling ICs to share data and computation in the cloud.
Behavioral Safety Research for Traffic Safety
During this project, HSRC will design a literature review to investigate knowledge related to pedestrian and bicycle safety in relation to interactions with automated vehicles (AVs) in order to identify and synthesize current literature and identify research gaps. The project team will develop relevant search topics and terms, and create a plan for searching and reviewing the literature in a comprehensive and objective way. The team will coordinate with project partners to conduct the review, develop an outline for the report, and create draft and final versions of the literature review/synthesis. Throughout the project, the team will coordinate with the project sponsor (NHTSA) and participate in project meetings (including a kickoff and final briefing), work planning, and progress reporting.
Bias Tracking and Reduction Methods for High-Dimensional Exploratory Visual Analysis and Selection
Exploratory visualization and analysis of large and complex datasets is growing increasingly common across a range of domains, and large and complex data repositories are being created with the goal supporting data-driven, evidence-based decision making. However, today's visualization tools are often overwhelmed when applied to high-dimensional datasets (i.e., datasets with large numbers of variables). Real-world datasets can often have many thousands of variables; a stark contrast to the much smaller number of dimensions supported by most visualizations. This gap in dimensionality puts the validity of any analysis at great risk of bias, potentially leading to serious, hidden errors. This research project developed a new approach to high-dimensional exploratory visualization that will help detect and reduce selection bias and other problems with data interpretation during exploratory high-dimensional data visualization. The project's results, including open-source software, are broadly applicable across domains, and have been evaluated with users in a health outcomes research setting. This offers significant potential to improve health care around the world.
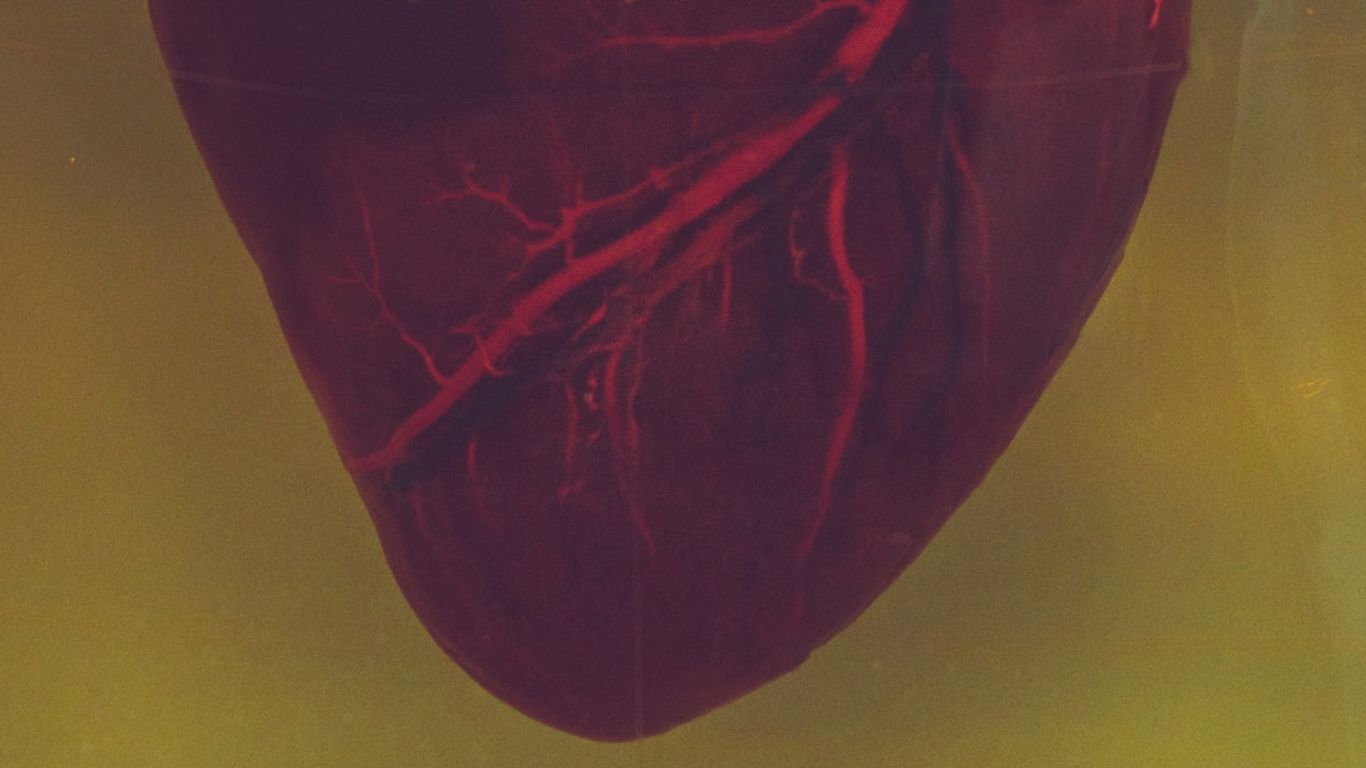
BioData Catalyst
NHLBI BioData Catalyst is a cloud-based ecosystem providing tools, applications, and workflows in secure workspaces. By increasing access to NHLBI datasets and data analysis capabilities, BioData Catalyst accelerates biomedical research that drives discovery, leading to novel diagnostic tools, therapeutics and prevention strategies for heart, lung, blood, and sleep disorders.
BioData Catalyst Coordinating Center (BDC3)
The BioData Catalyst Coordinating Center coordinates project management, communications, project reporting, and collaboration standards for the NHLBI BioData Catalyst project. BioData Catalyst is a cloud-based ecosystem providing tools, applications, and workflows in secure workspaces.
CC*IIE Integration: RADII: Resource Aware DatacentrIc Collaboration Infrastructure
CC*IIE Integration: RADII: Resource Aware DatacentrIc Collaboration Infrastructure - RADII will enable the creation and runtime management of end-to-end infrastructure tailored to support data-centric collaborations using networked Infrastructure-as-a-Service (IaaS).
CCMNet
Cyberinfrastructure (CI) has become ubiquitous in science and engineering research, partly due to the unprecedented acceleration and availability of high-performance computing (HPC) capabilities enabling many breakthrough discoveries. To advance scientific discovery, many researchers are faced with transitioning their work from local machines or departmental servers to large-scale CI, which requires developing new technical skills. Research computing facilitators, research software engineers, and other CI professionals (CIP) are an essential workforce needed to advise researchers on how to reshape the way they perform research, optimize workflows, educate and assist in the use of new technologies, and serve as information resources. However, it is a challenge for this community to keep abreast of the extensive array of tools, techniques, and specialized approaches needed to pursue today’s complex research questions. The CIP community relies on peer-to-peer knowledge sharing; this valuable knowledge is widely dispersed and difficult to find. A strong, collaborative mentor network is critical to successfully sustaining and advancing the CIP workforce to meet future scientific challenges. The Connect.Cl-based Community-wide Mentorship Network (CCMNet) is developing this network of subject matter experts from across the country, leveraging the Connect.Cl portal as the network's central coordination hub. Through these efforts, CCMNet is strengthening researchers’ and CIPs’ confidence in their abilities, enabling the exploration of new questions, and facilitating more rapid scientific discoveries and advancements.
The CCMNet program is building a mentor network that spans the broader CIP community by (a) developing a portal for making connections and exchanging knowledge, (b) forming partnerships with other mentor-centric programs in the community to advance support for all CIPs, (c) reaching out to under-served groups to create a more inclusive and diverse CIP community, and (d) developing best practices and guidance on mentorship for the benefit of the entire community. These efforts bring novel structure and consistency to the development of the CIP workforce, enabling a more advanced CIP workforce better able to support today’s research needs, as well as anticipate future needs.
Cell Cycle Browser (CCB) and TRACE (TRacking and Analysis of CEll Cycle)
The Cell Cycle Browser (CCB) is an interactive web tool for visualizing and simulating the human cell cycle to explore the timeline of molecular events during cell cycle progression. It was developed in the spirit of the UCSC Genome Browser, which provides a graphical view of genome sequence data along the physical coordinates of the chromosomes. Unlike the Genome Browser, however, the primary axis of the CCB browser is time. In its most basic functionality, the CCB displays time series data representing various molecular activities over the course of a single cell cycle. Single-cell traces from time-lapse fluorescent microscopy experiments—representing different biological activities from multiple cell lines—may be aligned in time to examine the temporal sequence of cell cycle events.
TRACE is an interactive web tool for tracking and segmenting cells from live-cell microscopy images.
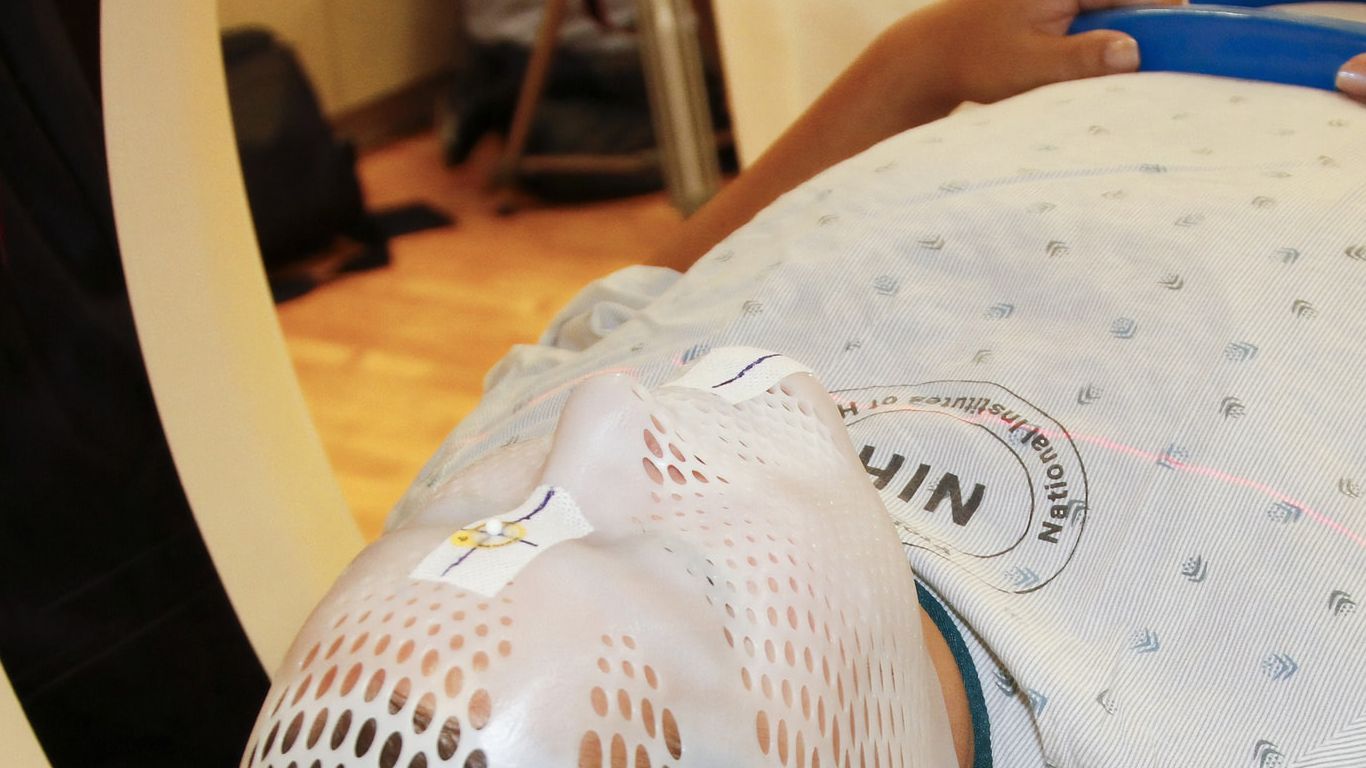
Center for Cancer Data Harmonization (CCDH)
The Center for Cancer Data Harmonization aimed to make the volumes of data arising from cancer research more accessible, organized, and powerful. CCDH worked with the National Cancer Institute's cloud-based data-sharing portal called the Cancer Research Data Commons. In the Commons, the goal is for disparate types of data generated by everything from basic science studies to clinical trials to be integrated and structured in ways that help researchers make advances and clinicians provide the best treatments. The center's work was organized around five key areas: community development, data model harmonization, ontology and terminology ecosystem, tools and data quality, and program management. RENCI contributed expertise in incorporating ontologies into tools for data validation, harmonization, and quality control.
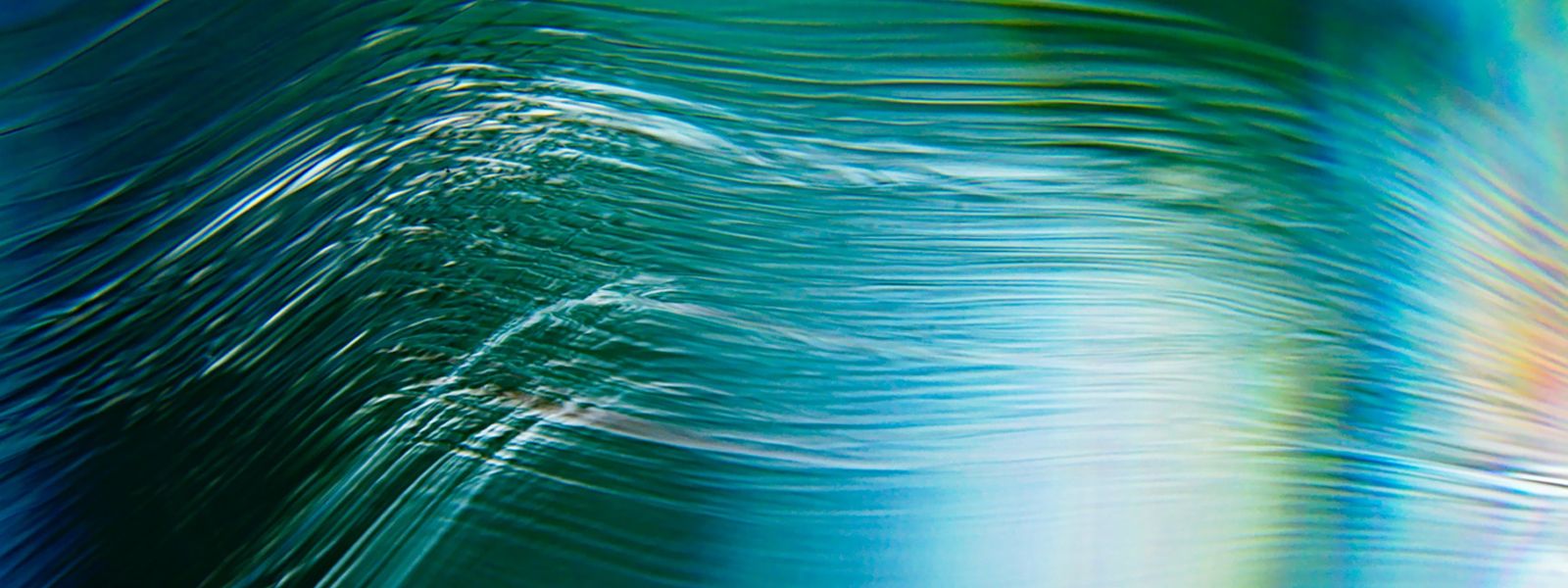
Chameleon Cloud
Chameleon is one of two mid-scale cloud testbeds funded by the NSFCloud program. It currently includes resources at the University of Chicago and the Texas Advanced Computing Center and consists of 650 multi-core cloud nodes, 5 petabytes of total disk space, and leverages 100 G/bps connections between the sites. Chameleon users are able to leverage large-scale and heterogeneous hardware, reconfigure the provisioned hardware partitions, and deploy their experiments on isolated partitions, free from interference from other experimenters. In order to broaden the set of experiments it supports, Chameleon is investing in experiments in reconfigurable Software Defined Networking (SDN) and higher bandwidth (40/100 Gbps) layer-2 WAN capabilities.
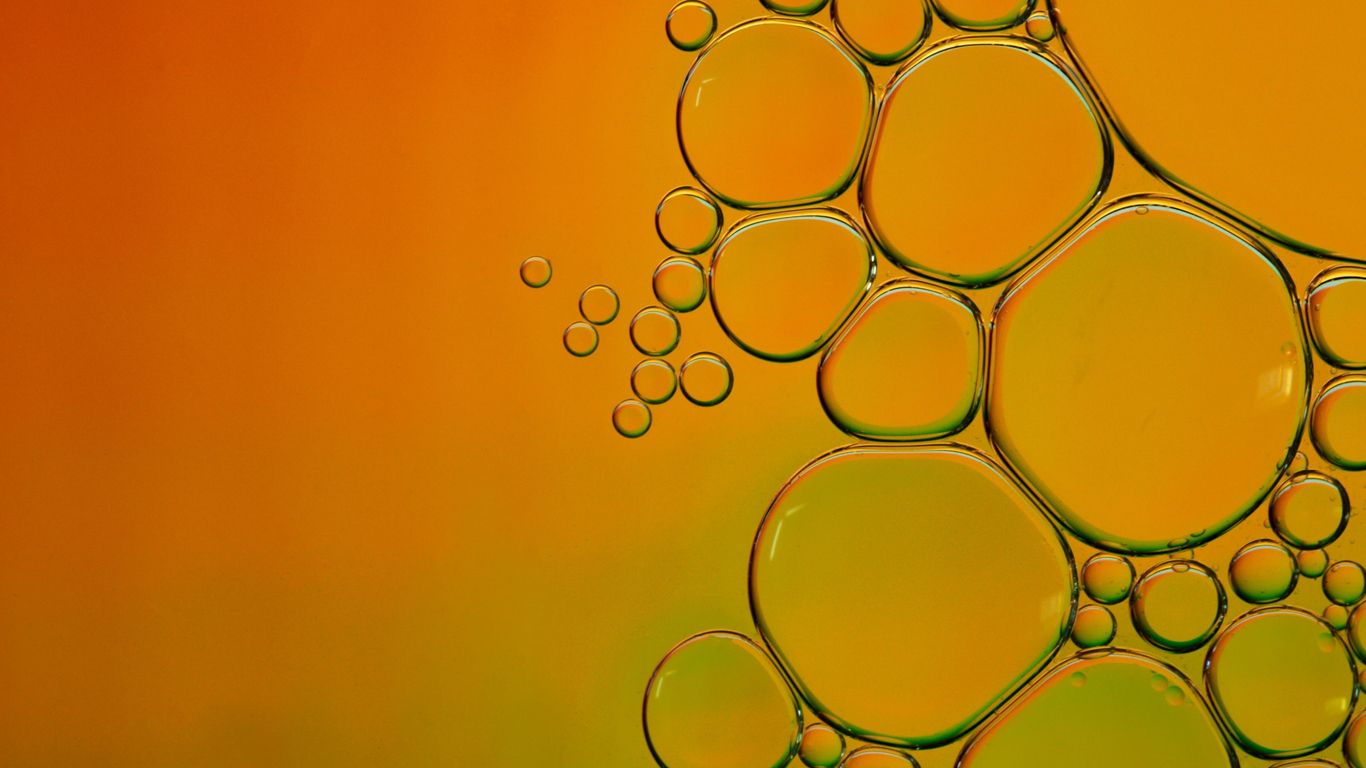
Chemotext2
Chemotext2 is a collaborative project with the UNC Eshelman School of Pharmacy seeking to develop a knowledgebase of potentially novel chemical-biological and entities-disease relationships, drawn from texts and abstracts in research journals. The project aims to extend the existing Chemotext database of similar relationships mined from abstracts that has been developed through a partnership between the School of Pharmacy and the UNC department of computer science. In addition to building a valuable science resource, Chemotext2 is an internally funded project that develops RENCI capabilities in knowledge networks and knowledgebases, text mining, machine learning, and ontologies. The work has helped to secure two related research grants: The Man to Molecule to Man project and the Data Translator project.
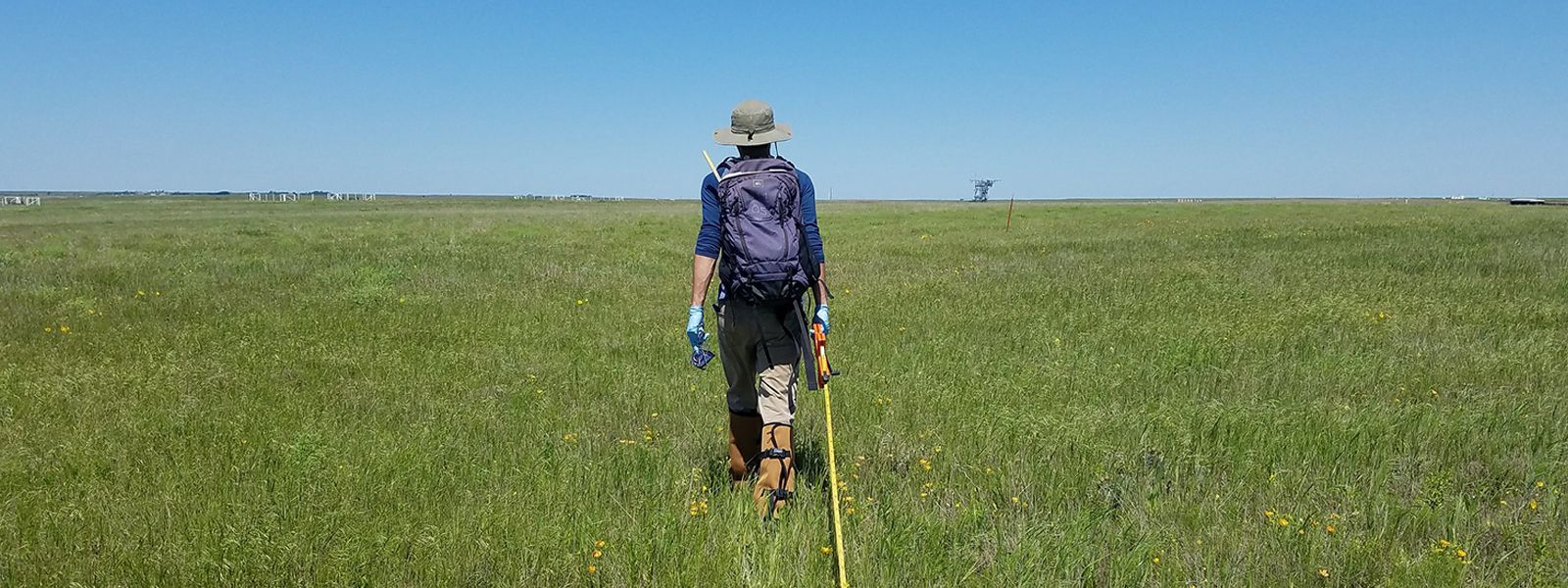
CI CoE Pilot
The Cyberinfrastructure Center of Excellence (CI CoE) pilot study aims to establish a reservoir of expertise on best cyberinfrastructure practices for the nation’s largest research facilities. The CI CoE pilot provides leadership, expertise, and active support to cyberinfrastructure practitioners at National Science Foundation Major Facilities and throughout the research ecosystem in order to ensure the integrity and effectiveness of the cyberinfrastructure upon which research and discovery depend. The CI CoE is a platform for knowledge sharing and community building; a partner for establishing and improving Major Facilities’ cyberinfrastructure architecture; and a forum for cyberinfrastructure sustainability, workforce development, and training.
CI CoE: CI Compass: An NSF Cyberinfrastructure (CI) Center of Excellence for Navigating the Major Facilities Data Lifecycle
CI Compass provides expertise and active support to cyberinfrastructure practitioners at NSF Major Facilities in order to accelerate the data lifecycle and ensure the integrity and effectiveness of the cyberinfrastructure upon which research and discovery depend.
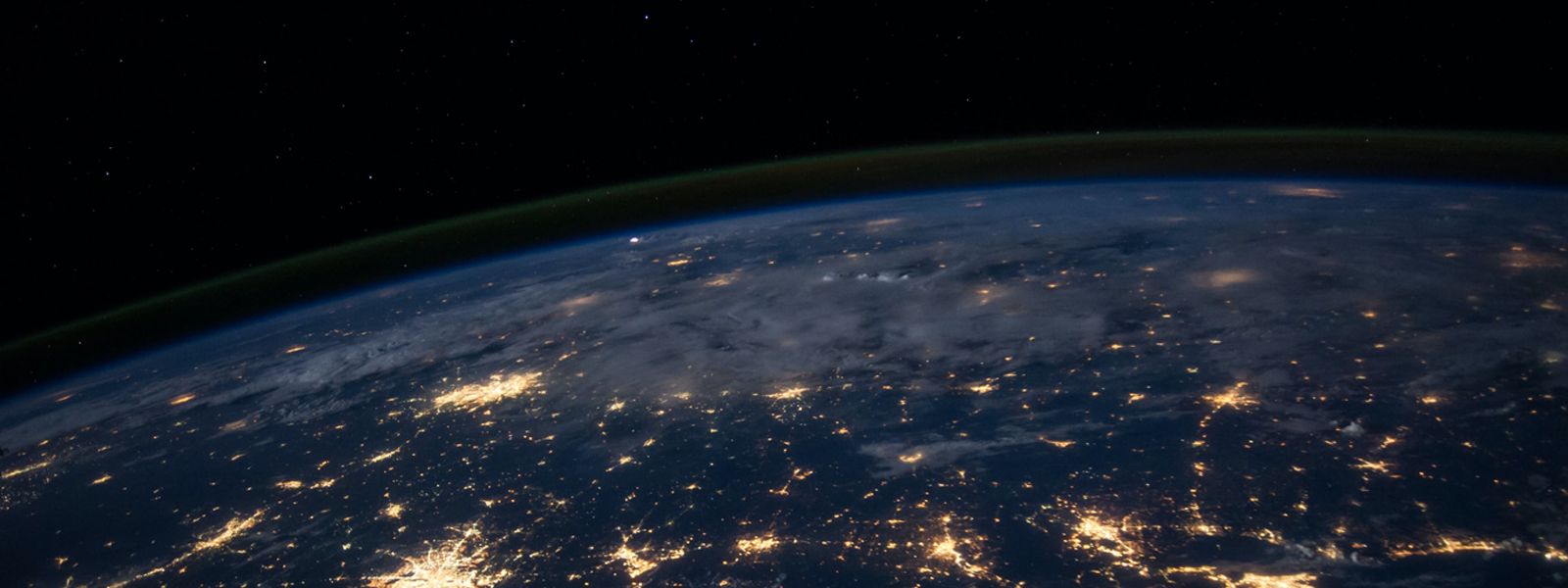
CICI: SSC: Integrity Introspection for Scientific Workflows (IRIS)
The Integrity Introspection for Scientific Workflows (IRIS) project aims to automatically detect, diagnose, and pinpoint the source of unintentional integrity anomalies in scientific workflows on distributed cyberinfrastructure. The approach is to develop an appropriate threat model and incorporate it in an integrity introspection, correlation and analysis framework that collects application and infrastructure data and uses statistical and machine learning (ML) algorithms to perform the needed analysis. The framework is powered by novel ML-based methods developed through experimentation in a controlled testbed and validated in and made broadly available on NSF production CI. The solutions are being integrated into the Pegasus workflow management system, which is already used by a wide variety of scientific domains. An important part of the project is the engagement with selected science application partners in gravitational-wave physics, earthquake science, and bioinformatics to deploy the analysis framework for their workflows, and iteratively fine tune the threat models, the testbed, ML model training, and ML model validation in a feedback loop.
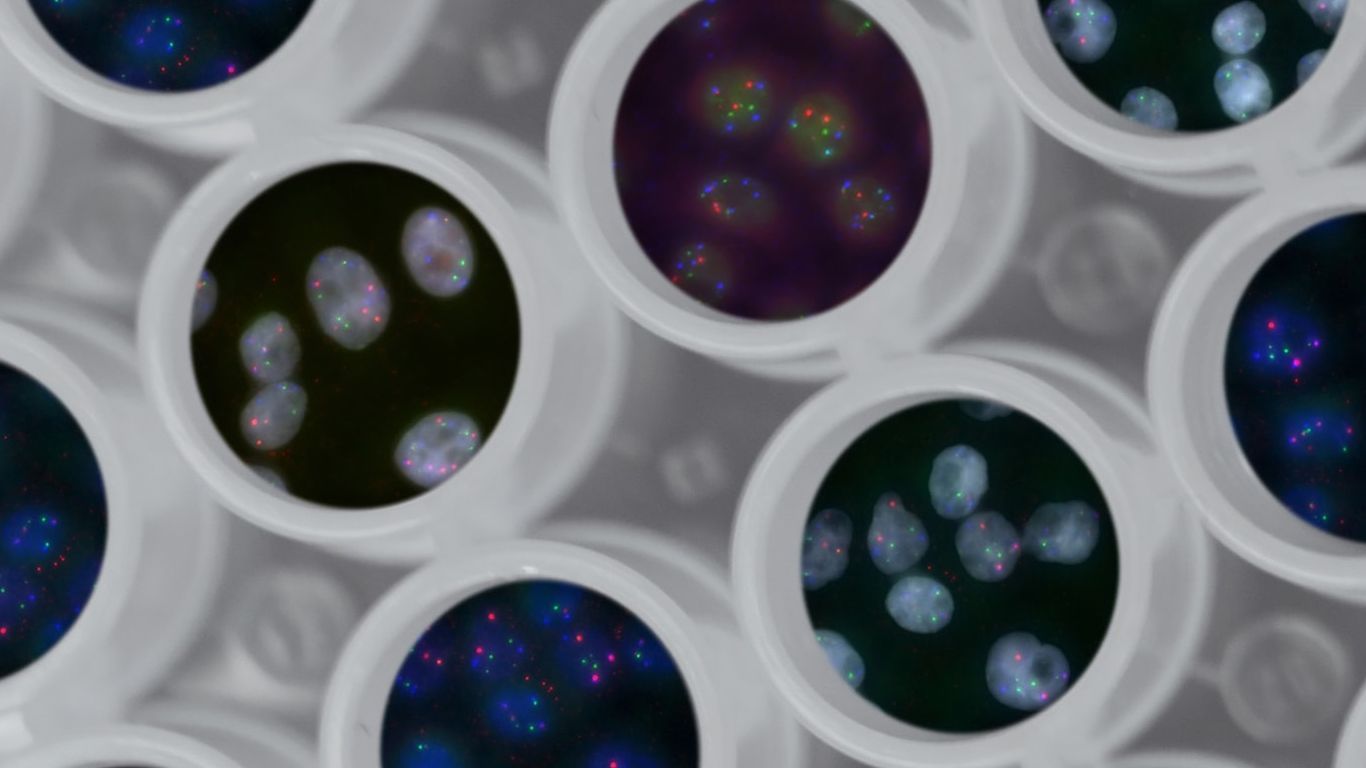
Clinical Trial Management Dashboard (CTMD)
RENCI is working with the Duke Clinical Research Institute’s Trial Innovation Center (TIC) on a project that will impact the collaborative capabilities between the CTSA sites. The TIC came to RENCI with a name-value-pair list of cross-team project proposal data with no easy method for parsing or generating insights. RENCI brought to bear the curative skills of trainees from the School of Information and Library Science (SILS) to inform a data model and ETL pipeline to transform these data into a structured database. RENCI is further using this database to collaboratively design an interactive analytical dashboard for visualizing the data and generating hypotheses about how to better support the team collaborations.
Coastal Hazard and Risk Modeling - AdcircLite-NC
Timely forecasting is crucial in the days and hours before a hurricane hits. AdcircLite-NC offers a rapid evaluation of storm surge and wave forecasts to aid decision makers both before and after a hurricane makes landfall along the North Carolina coast.
Coastal Hazard and Risk Modeling - Evacuation Modeling
To save lives, it is critical to know the best way to protect people in the path of a hurricane. While emergency managers use models to inform evacuation routes and timing, existing models are based primarily on “clearance time,” or ensuring that evacuees are on the roads for the shortest amount of time. The models do not take into account what populations are at most at risk, potential for injury or loss of life, or other social factors.
The storm surge and wave model ADCIRC forms the basis of a new comprehensive tropical cyclone impacts modeling system that integrates coastal oceanographic processes with mesoscale meteorological and hydrological processes to model water levels, winds, precipitation, and river drainage during emergency events. This comprehensive approach to modeling the hurricane-related hazards and uncertainties is then used in a state-of-the-art evacuation and sheltering model that optimizes for the aggregate risk to a coastal population, instead of only minimizing clearance times. The models are computed on RENCI supercomputers.
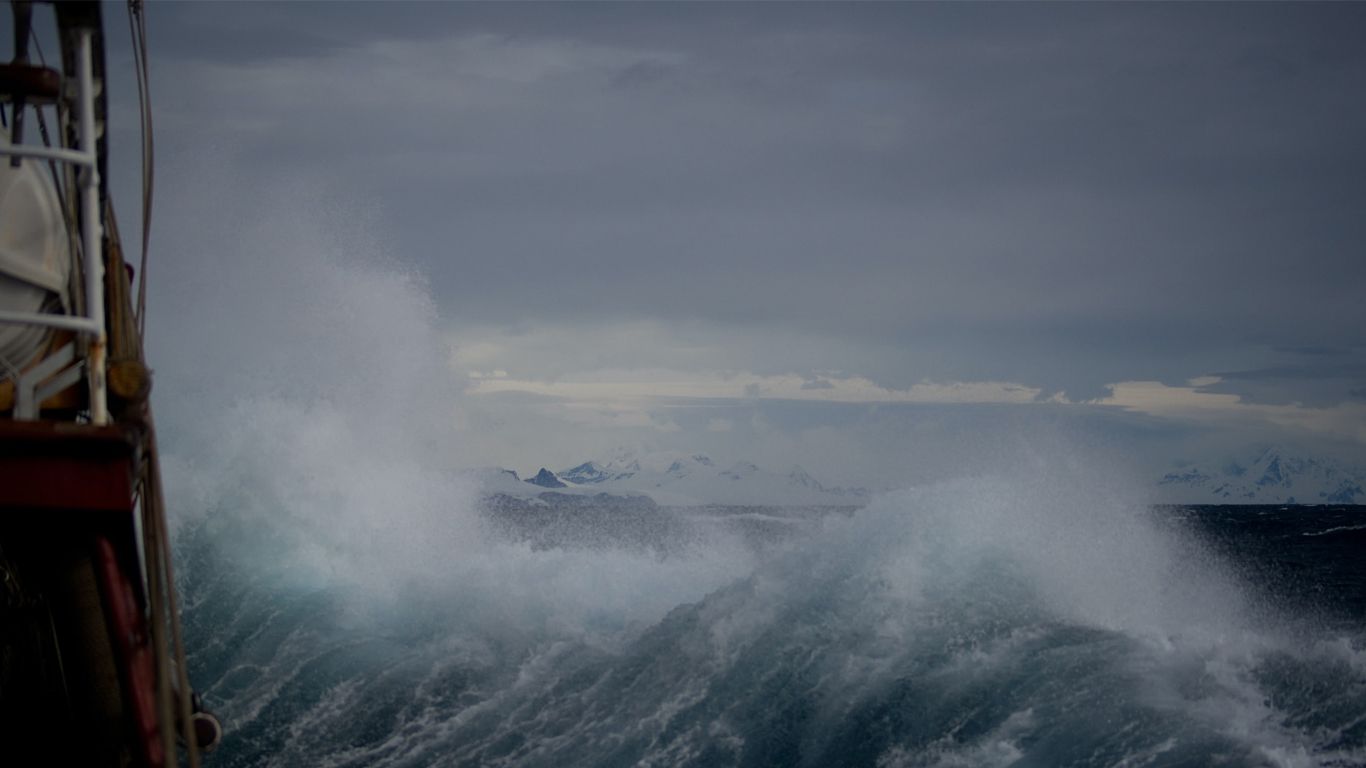
Coastal Hazards and Risk Research: Applications of the ADCIRC Storm Surge and Wind-wave Model
RENCI collaborates with Rick Luettich, director of the Institute of Marine Sciences and the DHS-funded Coastal Resilience Center, to support, advance, and extend research and applications of the ADCIRC storm surge and wind-wave model. Applications include probabilistic forecasting of storm surge levels during hurricanes, using AI and machine learning methods to simulate and predict hurricane tracks, and performance analysis and code tuning of ADCIRC software. ADCIRC is the core computational model in the ADCIRC Surge Guidance System (ASGS), a real-time software system that computes high-resolution coastal flooding predictions during tropical storms and hurricanes. The work was awarded a 2012 DHS Impact Award, a 2013 HPC Innovation Excellence Award, and a 2016 HPC Impact Award at the SC16 conference. ADCIRC users include the U.S. Coast Guard, Army Corps of Engineers, FEMA, and NOAA, several consulting firms, and hundreds of academic researchers worldwide.
Coastal Hazards, Equity, Economic prosperity and Resilience (CHEER) Hub
The Disaster Research Center at the University of Delaware has been awarded $16.5 million from the National Science Foundation to lead a multi-institutional effort exploring the tension and tradeoffs between a community’s goals of managing hurricane risk while also achieving equity and economic prosperity. The UD-led hub — Coastal Hazards, Equity, Economic prosperity and Resilience (CHEER) — is one of five NSF-funded projects announced recently as part of the agency’s Coastlines and People program, which is infusing $51 million in research funding to protect the natural, social and economic resources of U.S. coasts, and to help create more resilient coastal communities. R
Coastal Probabilistic Hazard Analysis (FEMA-BOA)
FEMA has recently conducted new coastal hazard and risk studies to support the mission and goals of the National Flood Insurance Program with new flood maps. These studies are costly and generate large volumes of model-generated data that capture the range of hurricane impacts for a region. The ADCIRC model has been used for all recent FEMA studies of this nature. Beyond the immediate use for mapping activities, these collections of model results can be used for other applications. The key to leveraging these data is to develop “surrogate models” that statistically represent the underlying model dynamics as best as possible, thus allowing for rapid statistical simulations of unmodeled events. This project will implement various surrogate modeling approaches using the flood insurance study for FEMA’s Region 3 (NC/VA border through the Delaware coast), which RENCI conducted. These data include time and spatially varying simulated waves, water levels, wind, atmospheric pressure, and currents, among other variables. These data could be useful for other efforts if they were more easily discoverable and accessible. The surrogate models will be developed and implemented by collaborators at the University of Notre Dame. RENCI will define and implement the needed cyberinfrastructure to make the data externally accessible through a geospatial database. The methods and approach will subsequently be used to develop similar databases for other regional FEMA coastal study datasets.
Conference on Data Science and Law
This one-day virtual conference (scheduled for April 12, 2021) and two-day in-person conference (Fall 2021) will bring together experts and researchers in law, data science, computer science, and social science with the goals of
- Building a community of law/data science/social science practitioners to develop a new research network;
- Highlighting the most promising avenues of research both for conference attendees and, perhaps via a Dear Colleague Letter and/or White Paper, for the larger interdisciplinary communities; and
- Keeping ethical decision making at the forefront in both law and data science.
Counterfactual-Based Supports for Visual Causal Inference
Data visualization is a critical and ubiquitous tool used to support data analysis tasks across a variety of domains. Visualizations are valued for their ability to “show the data” graphically, rather than using letters and numbers, in a way that enables users to assign meaning to what they see. This in turn helps users analyze complex data, discover new insights, make data-driven decisions, and communicate with other people about their findings. The correctness of these findings is therefore clearly contingent upon the correctness of the inferences that users make when viewing or interacting with a data visualization tool. However, recent studies have shown that people often interpret visualized patterns as indicators of causal relationships between variables in their data even when no causal relationships exist. The result is that visualizations can dramatically mislead users into drawing erroneous conclusions. This project develops a new approach to visualization, based on the concept of counterfactual reasoning, designed to help users draw more accurate and generalizable inferences when analyzing data using visualization tools. The project's results, including open-source software, are intended to be broadly applicable across domains. In addition, the project will be evaluated with data and users in the population health domain with the potential to contribute to improvements to human health.
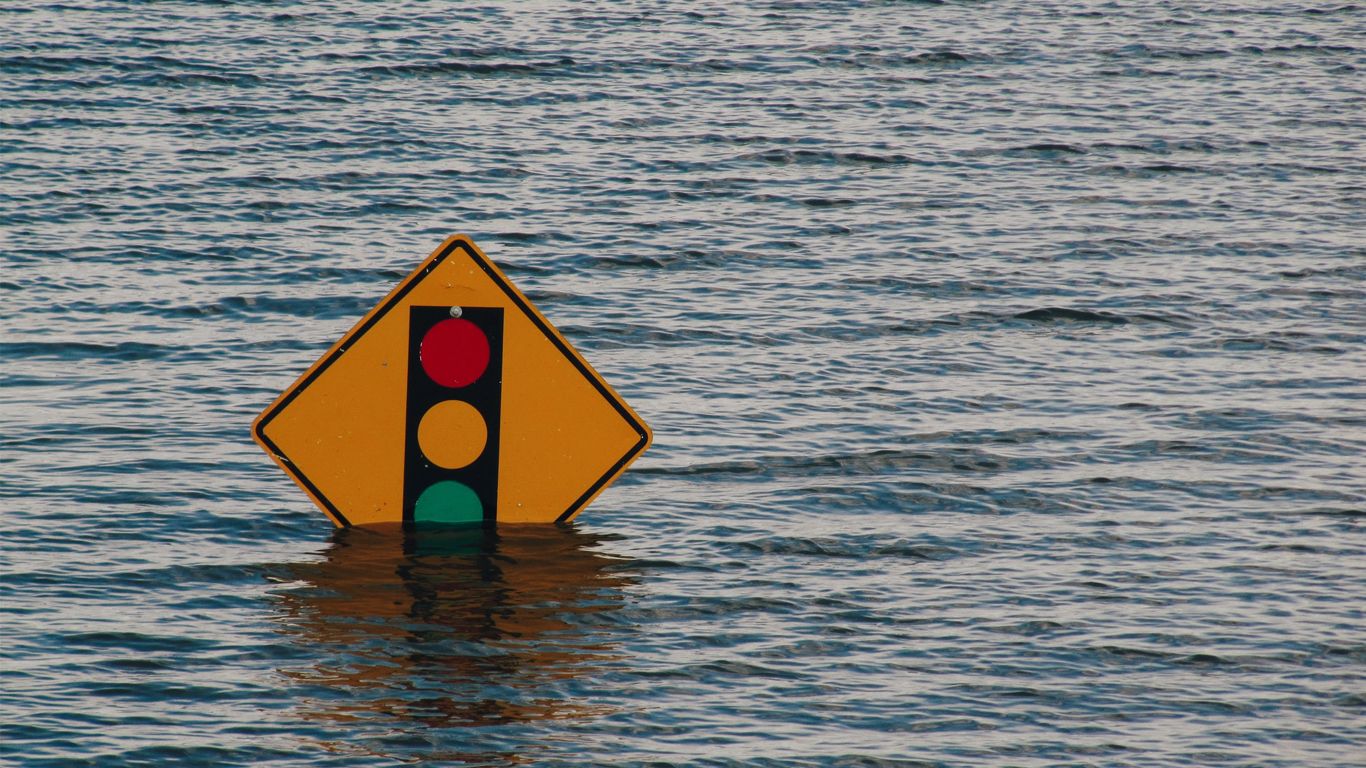
Coupling the National Water Model to the Coastal Ocean for Predicting Water Hazards
This NOAA Integrated Ocean Observations System/Coastal Ocean Modeling Testbed project is examining the connection between inland/riverine flooding and coastal water levels as they interact in the middle area where both are important to overall inundation extents and depths. The middle zone, in which these two flooding components interact, is not currently well modeled. This is an active area of research, principally because of the increasing co-occurrence of hurricane-driven storm surge and precipitation-related riverine runoff, called “compound flooding”. The project will specifically establish a testbed for different approaches to this model coupling problem. The coastal model used in this project is the ADCIRC storm surge, tide, and wind-wave model. Several upland/hydrologic/hydraulic models are being studied, including the NOAA National Water Model and the US Army Corps model Gridded Surface Subsurface Hydrologic Analysis (GSSHA). The primary test case is of Hurricane Florence (2018), which caused extensive damage from riverine flooding and had several locations where compound flooding is demonstrable.
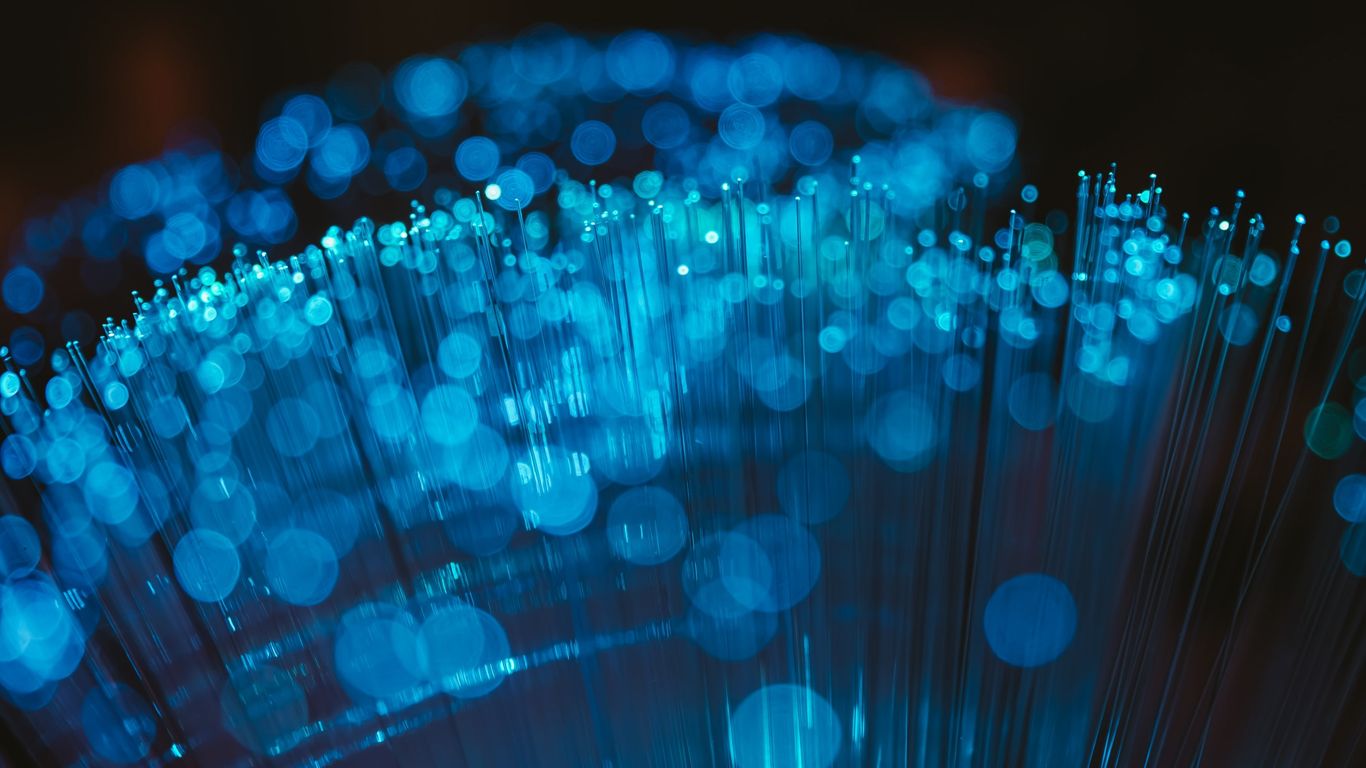
Creating Dynamic Superfacilities the SAFE Way (CICI SAFE)
The term “superfacility" describes an integration of two or more existing Department of Energy facilities that use high performance networks and data management software in order to increase scientific output. Currently, superfacilities are manually built for a specific scientific application or community, which limits their uses to large, long-lived projects. The SAFE Superfacilities project brings together researchers and IT support organizations from RENCI, UNC-Chapel Hill, Duke University, and the DOE’s Energy Sciences Network (ESnet) to develop a way to create dynamic superfacilities on demand. The project puts to use advances in campus science networks (Science DMZs) and federated Infrastructure-as-a-Service in an effort to generalize and automate the creation of superfacilities while overcoming their security challenges. By design, superfacility dynamic network links bypass campus security appliances in order to maintain a friction-free network path; security for these paths is typically addressed by managing interconnections manually.
Critical-Zone Collaborative Network (CZCN Hub)
The goal of the Critical-Zone Collaborative Network is to establish an adaptive and responsive research network that supports investigations of the Earth’s Critical Zone (CZ). This network will consist of two components that will work together to advance knowledge, education, and outreach in this convergent science: 1) Thematic Clusters of fixed or temporary locations will conduct basic research on significant, overarching scientific questions concerning the structure, function, and processes of the Critical Zone. These U.S.-based Clusters could include existing observatories engaged in collecting environmental data, other monitoring locations that have been in operation for extended periods of time, and new sites that will support the scientific goals of the Cluster; 2) A Coordinating Hub that will oversee the compatibility and archiving of the data resulting from the Thematic Clusters, coordinate outreach and community-building activities, support the use of network facilities by outside researchers, and plan for infrastructure needs of the network.
Data Assimilation Development and Arctic Sea Ice Changes
The assimilation of data into physically-based computational models has emerged as one of the central themes of research in applied mathematics over recent years. The development of effective and efficient data assimilation (DA) schemes raises a number of fundamental challenges. This proposal will pick up this challenge with two contrasting, but interrelated directions of research:
A. Designing coherent structure based approaches to ocean DA. B. Developing DA schemes designed specifically to work with next generation sea-ice models.
The first direction (A) constitutes a new approach to dealing with the fundamental conundrum of DA, namely that the two basic strategies, coming from optimization and statistics, work well for high-dimensional and nonlinear systems respectively. But neither approach works effectively for both. The new approach being developed here will initially be focused on the all-important issue of assimilating data from Lagrangian observations of the ocean. The idea rests on the extensive past work, supported by ONR, on Lagrangian Coherent Structures.
The goal of the second direction (B) will be to bring advanced DA schemes to bear on the problem of determining how and when Arctic sea-ice will break up. The changes precipitated by a warming Arctic Ocean are already evident and it will be critical in the coming years to understand, and be able to predict, the location of open water and the presence of degrading ice. It is only the next generation sea-ice models, which account for material properties of the ice, that will be in apposition to properly capture these events. At the same time, data from observations will be critical to guide and correct these models. DA for these models, however, poses a fundamental challenge as the underlying numerical solvers are both Lagrangian and adaptive-mesh based.
The full picture of Arctic sea-ice brings up other issues attendant to the overall dynamics of the Arctic. These include the following topics to be considered as part of this overall effort: (i) the degrading of the ice pack by waves in the marginal ice zone, (ii) how much cold water is transported at depth away from the Arctic, which is believed to be measurable as outflow through a small number of chokepoints surrounding the Arctic basin, and (iii how warm water, originating in the tropics, is transported northward and such meteorological features as hurricanes are thought to play a key role.
Data Bridge for Neuroscience: A novel way of discovery for Neuroscience Data
Neuroscience is at an inflection point where more and more data are being aggregated and shared through common repositories. The main challenge facing the neuroscience community in the Big Data era is the difficulty of discovering relevant datasets across these repositories. The complication of effective discovery and identification of relevant data forms the last mile problem for long tail of science data. Solving this problem can increase the value of the data through reuse and repurposing and can immensely benefit the NSF Brain Initiative by providing increased access to data in its various thematic areas. This project will initiate studies on the application of a novel data discovery system for Neuroscience based upon a platform called DataBridge, which the project team has developed under a grant from the NSF Big Data program. DataBridge applies "signature" and "similarity" algorithms to semantically bridge large numbers of diverse datasets into a "sociometric" network. The DataBridge for Neuroscience platform will attempt to harness complex analytics algorithms developed by neuroscience researchers in order to extract key signatures and find data associations from large volumes, and diverse collections, of so-called "long-tail" neuroscience data. By providing a venue for defining complicated search criteria through pattern analysis, feature extraction and other relevance criteria, DataBridge provides a highly customizable search engine for scientific data. This project will conduct a preliminary, feasibility study on the applicability of DataBridge on Neuroscience data with two goals: 1. Implement a pilot DataBridge system for Neuroscience and demonstrate a proof of concept for semantically bridging a small collection of neuroscience datasets, and 2. Conduct a workshop to develop a coalition of users from the neuroscience community in order to build a sustainable DataBridge-based infrastructure for neuroscience. This community-based activity will leverage the community infrastructure created by the NSF Big Data Hubs and Spokes program.
Data Commons
The goal of this project is to establish an NIH Data Commons, a shared virtual space where scientists can work with the digital objects of biomedical research such as data and analytical tools. The NIH Data Commons Pilot Phase Consortium (DCPPC) will test ways to store, access, and share biomedical data and associated tools in the cloud so that they are FAIR (Findable, Accessible, Interoperable and Reusable). In phase I, awardees are to design innovative solutions that meet the needs of one or more of the computational, data, and scientific key capabilities of the Data Commons. All awardees work collaboratively as a consortium toward achieving NIH’s comprehensive vision for a Data Commons. Each of the eight Key Capabilities (KCs) addresses specific challenges faced by scientists working with large-scale biomedical data. Each KC has unique objectives and deliverables in the form of stand-alone Minimum Viable Products (MVPs).
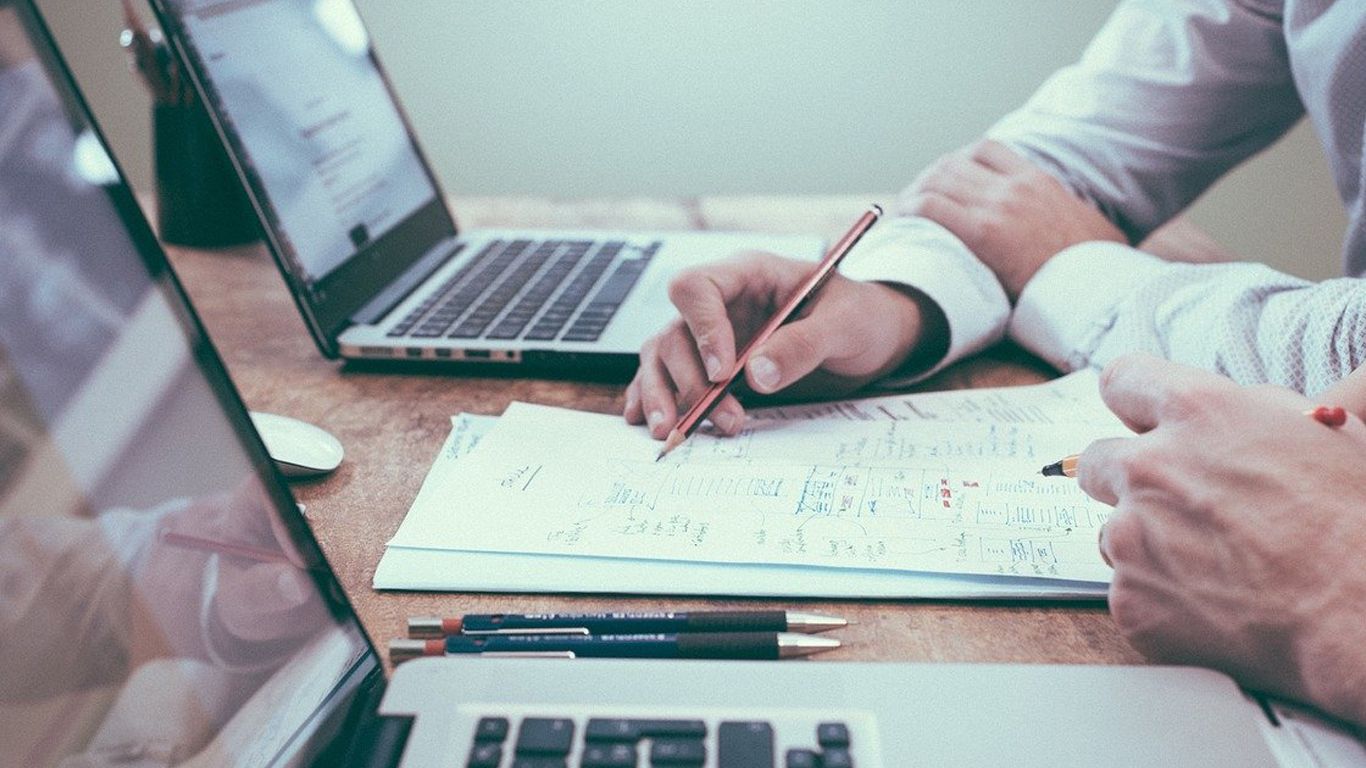
Data Matters
Data Matters™ is a week-long series of one and two-day courses aimed at students and professionals in business, research, and government. The short course series gives students the chance to learn about a wide range of topics in data science, analytics, visualization, curation, and more from expert instructors.
Data Translator ARAGORN
The NCATS Biomedical Data Translator program applies semantic integration strategies to share chemical, genetic, phenotypic, disease, ontological, and other ‘knowledge sources’. Taken together, the >300 knowledge sources form an integrated data ecosystem and technology platform—the Translator system—to support clinical and translational science. RENCI contributes to three Translator Projects, including the Ranking Agent project team. The Ranking Agent team has produced a tool called ARAGORN (Autonomous Relay Agent for Generation Of Ranked Networks), which represents the next iteration of its successful ROBOKOP tool. ARAGORN answers user-specified biomedical questions by reasoning over a federated knowledge graph and applying on-the-fly ranking to produce the most accurate results quickly. Additionally, ARAGORN attempts to bridge the precision mismatch between data representations and algorithms that require specificity and users who pose questions and prefer answers at a more abstract level. ARAGORN uses elements of specific answers to create gestalt explanations, clustering, and combining answers with similar content, thereby revealing the commonalities and contradictions in answers. As development continues, the Ranking Agent team will formulate and validate new graph-based methodologies for determining the best answers to user-asked biomedical questions.
Data Translator CAM/AOP Knowledge Provider
The NCATS Biomedical Data Translator project applies semantic integration strategies to share chemical, genetic, phenotypic, disease, ontological, and other ‘knowledge sources’. Taken together, the >300 knowledge sources form an integrated data ecosystem and technology platform—the Translator system—to support clinical and translational science. RENCI contributes to three Translator Projects, including the Exposures Provider Service. The CAM/AOP Knowledge Provider is a subproject of the Exposures Provider which explores integration of Causal Activity Models (CAMs) into the Translator knowledge graph. CAMs are small knowledge graphs modeled using the OWL Web Ontology Language, pioneered by the Gene Ontology project. An OWL reasoner is used to augment the CAM knowledge graphs with contextual information provided by reference ontologies. The CAM/AOP knowledge provider includes a datasets of CAMs provided by the Gene Ontology and Reactome projects, and we are also exploring publication of Adverse Outcome Pathways (AOPs) using this approach.
Data Translator Standards and Reference Implementations
The NCATS Biomedical Data Translator program applies semantic integration strategies to share chemical, genetic, phenotypic, disease, ontological, and other ‘knowledge sources’. Taken together, the >300 knowledge sources form an integrated data ecosystem and technology platform—the Translator system—to support clinical and translational science. RENCI contributes to three Translator Projects, including the Standards and Reference Implementations (SRI) team. This team is responsible for producing a suite of standards and tools, a model for their governance, and processes to coordinate the integration of Translator teams and the shared implementation of tools and standards:
- Community governance coordination aims to develop software with community buy-in in order to ensure an effective collaboration environment and drive consortium-wide consensus on other Translator components.
- Architecture and API specifications drive community efforts to define details of project architecture and communication protocols for program components.
- The Biolink model defines the standard entity types, relationship types, and schema shared by all Translator components, including related utility libraries and a novel approach to accommodate flexible data modeling perspectives.
- Integrated reference ontologies provide Biolink-compliant terms and relationships.
- A continually-updated knowledge graph provides Translator with a standardized and integrated global view of the whole information landscape.
- Next-generation shared Translator services aim to remove integration barriers by providing services to support validation, lookup, and mapping functionality for use across Translator.
Data Translator: ICEES+ Knowledge Provider
The NCATS Biomedical Data Translator project applies semantic integration strategies to share chemical, genetic, phenotypic, disease, ontological, and other ‘knowledge sources’. Taken together, the >300 knowledge sources form an integrated data ecosystem and technology platform—the Translator system—to support clinical and translational science. RENCI contributes to three Translator Projects, including the Exposures Provider Service, which has developed ICEES (Integrated Clinical and Environmental Exposures Service) as a regulatory-compliant framework and approach for openly exposing and sharing integrated clinical and environmental exposures data. ICEES was designed as a general approach to overcome the numerous regulatory, cultural, and technical challenges that hinder efforts to openly share clinical data or any data containing personally identifiable information (PII). ICEES is currently being used to support use cases on asthma and related common pulmonary diseases, primary ciliary dyskinesia and related rare pulmonary diseases, coronavirus infection, and drug-induced liver injury. ICEES allows user to pose questions such as: are there racial disparities in the impact of exposure to airborne pollutants on asthma? what medications are associated with good/poor outcomes, and do these relationships vary by demographic subsets of patients? how do socioeconomic factors interact with airborne exposures to influence rates of asthma-related ED visits? While ICEES provides a valuable open clinical resource in and of itself, when coupled with the Translator system, ICEES provides an even more powerful open resource that allows users to pose questions such as are there drugs that can be repurposed to treat DILI-associated phenotypes or outcomes, or allow a patient to continue on a drug that is otherwise effective? ICEES is under continual development, with new use cases and analytic features planned.
DataBridge
DataBridge, a collaboration between RENCI and the School of Information and Library Science, develops tools and methods to extract and use knowledge from the millions of data sets created by thousands of scientists worldwide. The ability to discover and use these data sets across disciplines is one way to accelerate progress in science and engineering. DataBridge works as an interface similar to social networking sites that show relationships among data points and enables access to a wide range of research databases and links to data sets. Funded by the NSF, DataBridge received additional NSF support to build a DataBridge for neuroscience research under the title, “EAGER: Data Bridge for Neuroscience.”
DataNet Federation Consortium
The DataNet Federation Consortium infrastructure enables collaboration environments for multi-disciplinary science and engineering initiatives. Within each initiative, data management infrastructure organizes data into sharable collections. The collections are organized into national data infrastructure through federation of the independent data grids. Collaborations are sustained through a community-based collection life cycle for science and engineering data.
Development: The Next-Generation Public Charging Infrastructure and Cyber-Information Network for Enhanced Inclusion and Independent Living of Power Mobility Device Users
This project pilots a public charging infrastructure and cyber-information system to support the outdoor use of power mobility devices (PMDs), to improve the mobility and inclusion of their owners. People with disabilities use PMDs such as power wheelchairs and electric scooters to improve their mobility, but users and caregivers consistently report the energy constraints of batteries as one of the main reasons for limited away-from-home mobility. The project objectives are to: (1) design, develop, and test a pilot public physical charging network accessible for PMD charging; (2) make the charging stations real-time Internet of Things (IoT)-connected through Google Maps services; (3) build smart energy monitoring hardware to track the PMD energy consumption; (4) develop a cloud-based, energy consumption prediction algorithm to enable route planning;( 5) write a Best Practice Protocol to enable the charging network scaling up; and (6) increase the awareness of the general population regarding the needs of people with disabilities and aging adults. The outcomes are: (1) the PMD users will be able to successfully use public charging stations and charging apps; (2) increase the overall distance traveled by PMD users by 10%; (3) increase the average participation of outdoor miles in total traveled distance; and (4) the life-time of PMD batteries will increase. Project outputs include: (1) a pilot charging infrastructure in Chapel Hill, (2) a charging app for managing the charging process, (3) cloud-located AI-based software for PMD energy consumption estimation, and (4) Best Practice Protocol to guide charging network expansion.
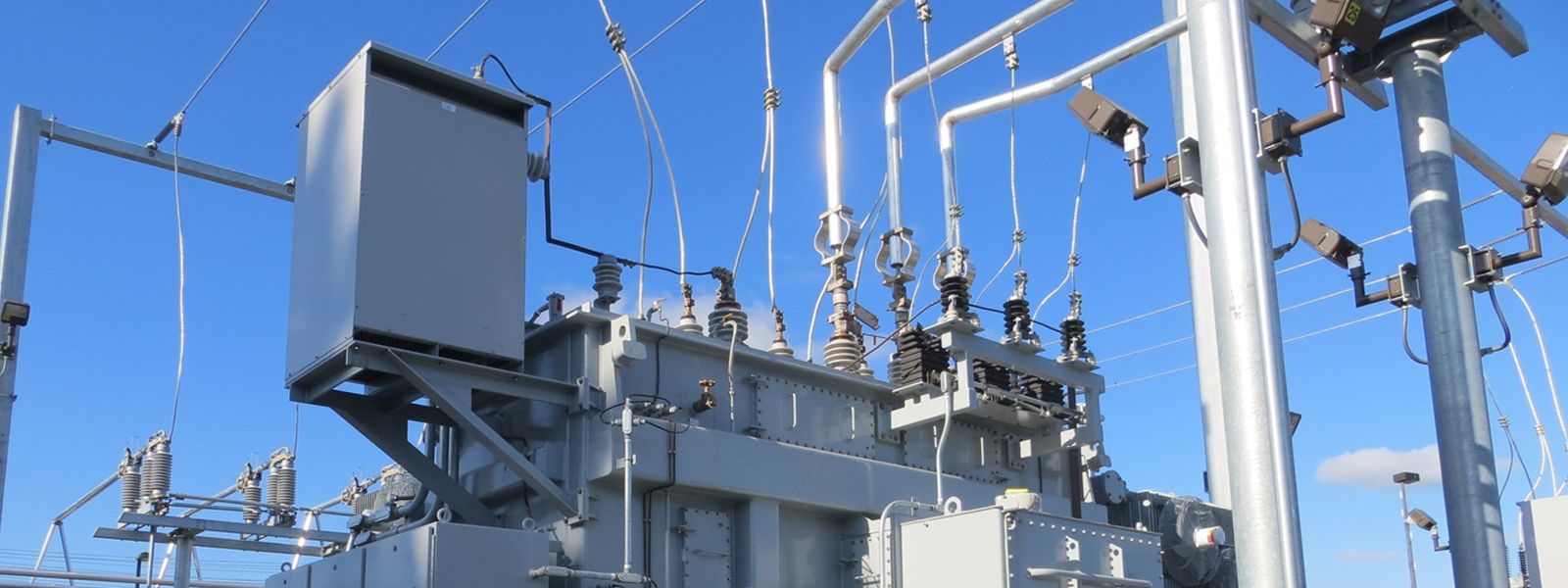
Distinct: A Distributed Multi-Loop Networked System for Wide-Area Control of Large Power Grids
Distinct aims to improve the robustness and reliability of electric power systems by creating a distributed multi-loop networked system for wide-area control of large power grids.
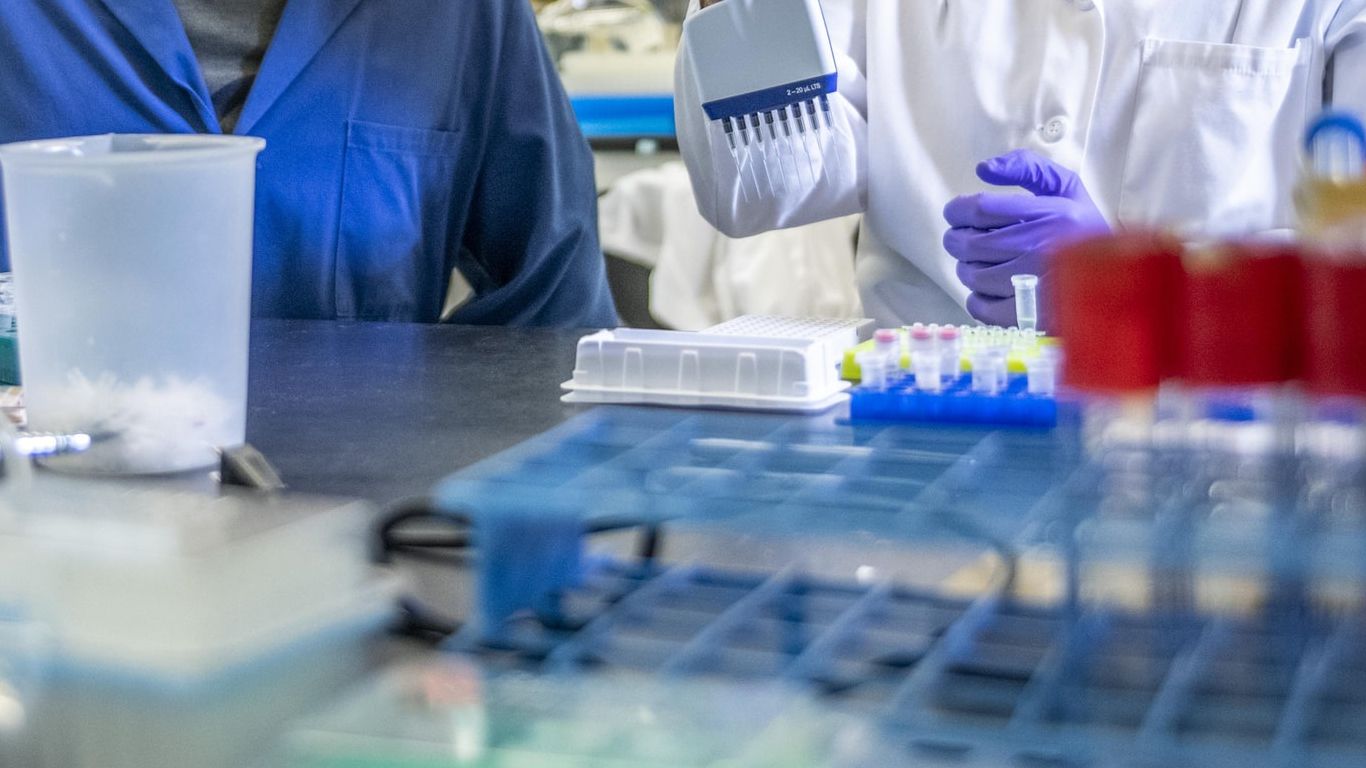
Drug Repurposing for Cancer Therapy: From Man to Molecules to Man
From Man to Molecules to Man, or M^3, is a NIH BD2K award to the UNC Eshelman School of Pharmacy with RENCI as a collaborating partner. M^3 seeks to identify novel drug uses through a pipeline that incorporates data on the relationships between drugs and their side effects (extracted from social media and various health forums), QSAR modeling data, and knowledge bases on chemical-biological and entity-disease relationships.
Dug Semantic Search
Dug is a flexible, scalable, semantic search tool for biomedical datasets that leverages emerging knowledge graph-based methods to intelligently suggest relevant connections derived from peer-reviewed research. Developed through the National Heart, Lung, and Blood Institute’s (NHLBI) BioData Catalyst (BDC) platform, Dug can efficiently ingest, index, and search over nearly any type of study metadata, and utilizes evidence-based relationships from curated knowledge graphs to explain why certain results are being returned. The Dug search engine is publicly available as an open source package to provide the biomedical community with a powerful, flexible tool for searching intuitively across an increasingly sprawling public data landscape.
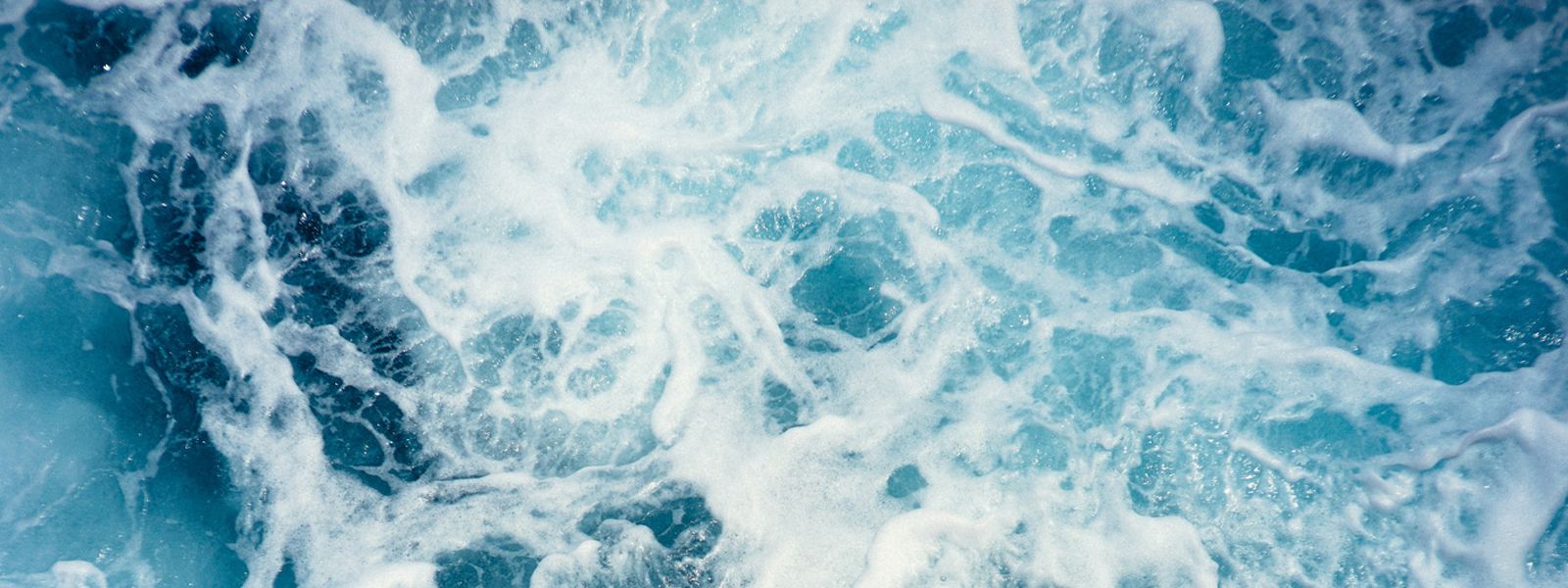
DyNamo
Scientists face numerous challenges when integrating data from multiple scientific instruments and data stores into their workflows. The Delivering a Dynamic Network-Centric Platform for Data-Driven Science (DyNamo) project helps increase scientific productivity through a platform that manages high-performance data flows and coordinates access to cyberinfrastructure.
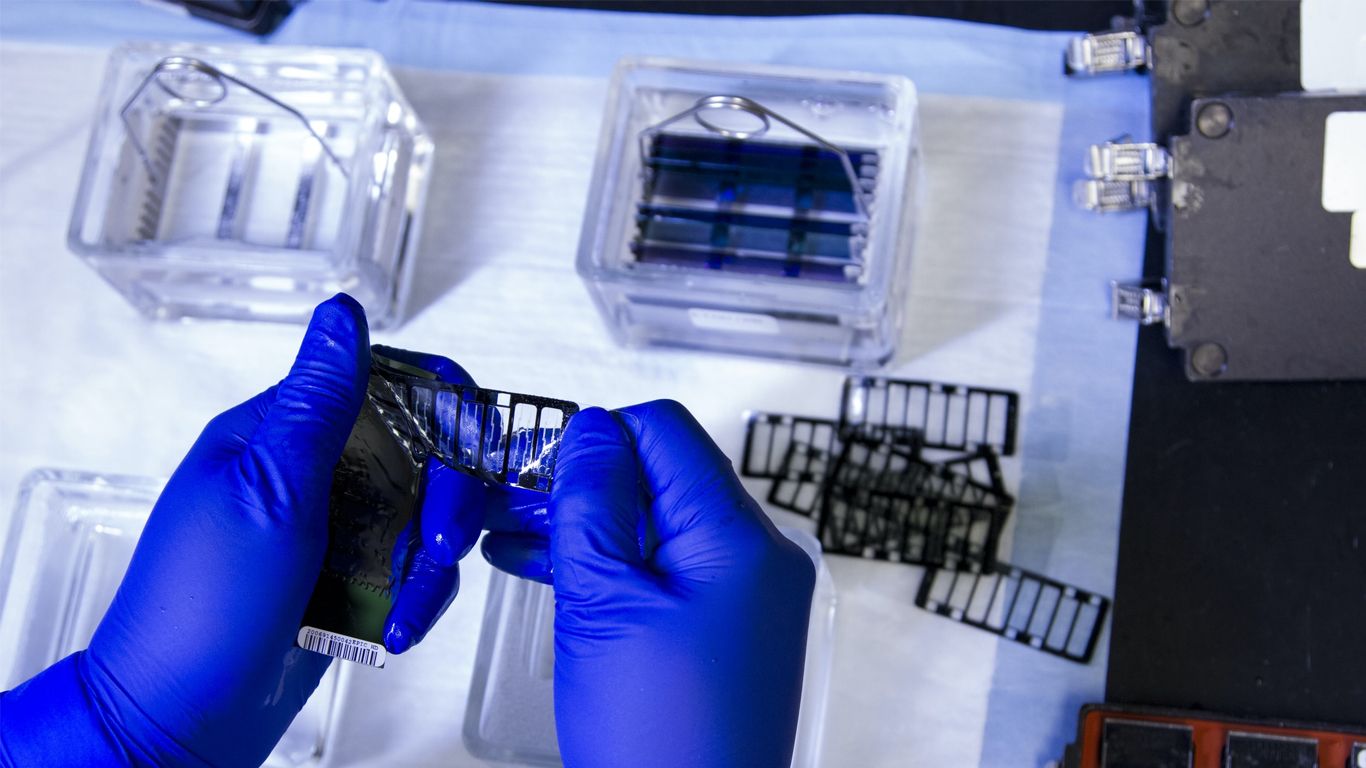
Enabling machine-actionable semantics for comparative analyses of trait evolution (SCATE)
We aim to enable comparative trait analyses that are powered by computational inferences and machine reasoning based on the meanings of trait descriptions. We will do this by addressing three long-standing limitations in comparative studies of trait evolution: recombining trait data, modeling trait evolution, and generating testable hypotheses for the drivers of trait adaptation. We will create a centralized computational infrastructure that affords comparative analysis tools the ability to compute with morphological knowledge through scalable online application programming interfaces (APIs), enabling developers of comparative analysis tools, and therefore their users, to tap into machine reasoning-powered capabilities and data with machine-actionable semantics. To accomplish this, the project will adapt key products and know-how developed by the Phenoscape project, including an integrative knowledgebase of ontology-linked phenotype data (the Phenoscape KB), metrics for quantifying the semantic similarity of phenotype descriptions, and algorithms for synthesizing morphological data from published trait descriptions.
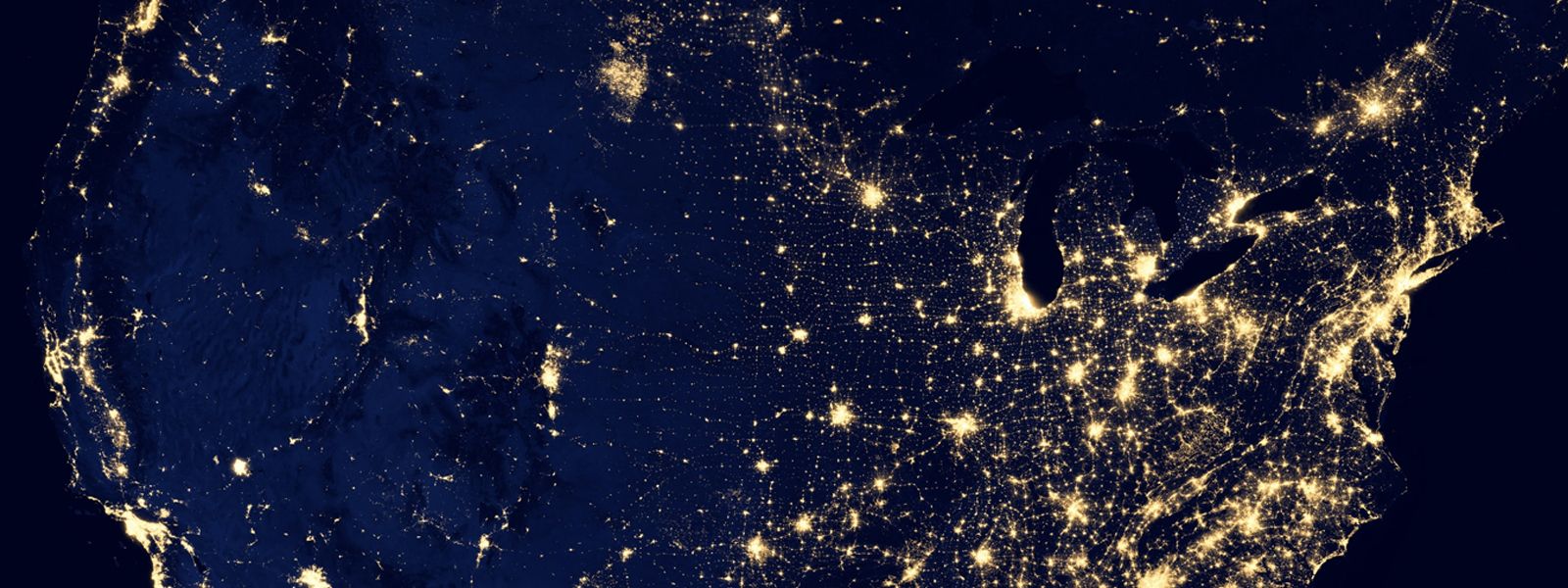
ENTeR
Enabling Network Research and the Evolution of a Next Generation Midscale Infrastructure (ENTeR) helps the computer and information science and engineering (CISE) community prepare to transition to next-generation mid-scale research infrastructure while ensuring existing research infrastructure remains available and relevant during the transition.
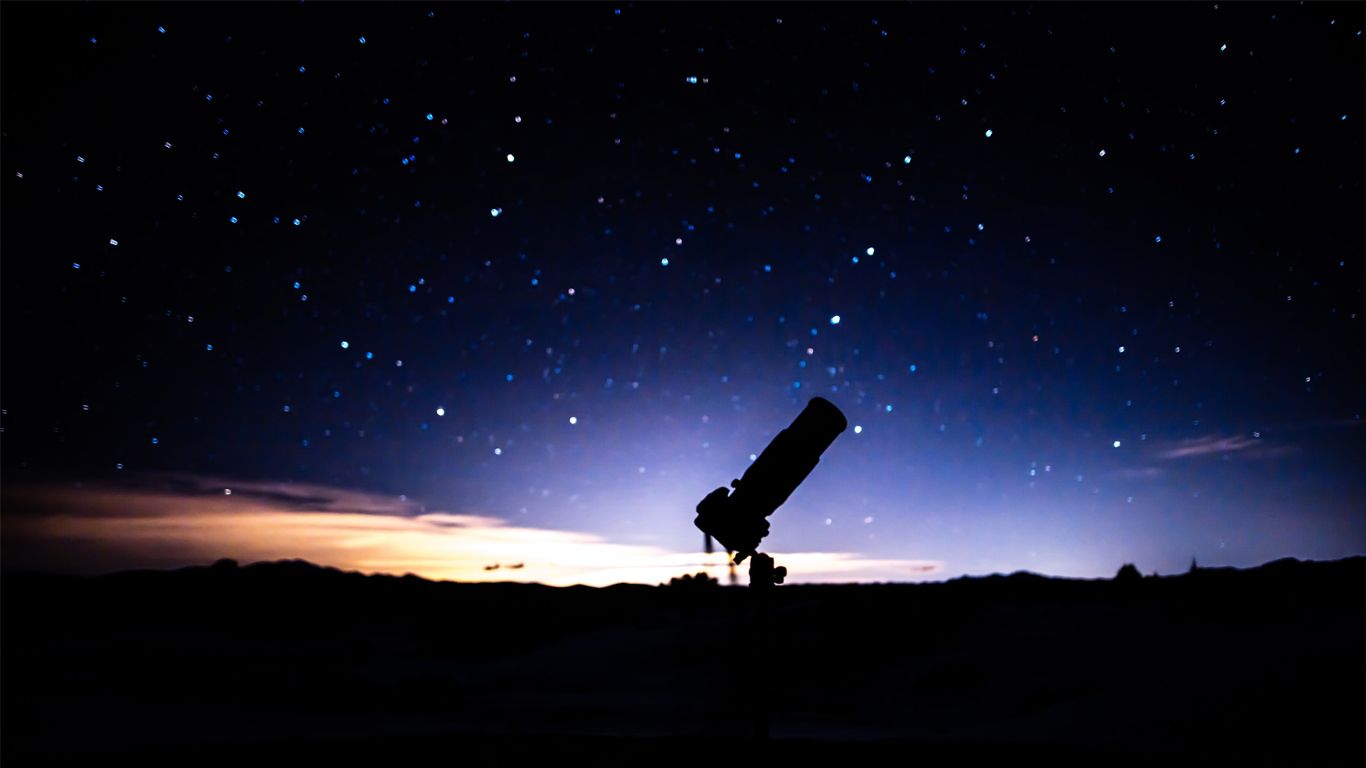
Evryscope
The Evryscope (“wide-seer”) is an array of telescopes pointed at every part of the accessible sky simultaneously and continuously, together forming a gigapixel-scale telescope monitoring an overlapping 8,000 square degree field every two minutes. Operating at CTIO since May 2015, Evryscope is being developed at UNC-Chapel Hill by Nick Law, assistant professor of physics, and his team. RENCI has worked closely with the team to use iRODS as the data management and transfer platform to examine image data taken from the telescope. The researchers hope to receive funding from the NSF to support the full development of the design. The proposal envisions collecting approximately one petabyte of image data over three years. It also envisions creating a data store that astrophysicists can query, which could be as large as 90 terabytes. Analytics on the image data will be used to detect transiting exoplanets and transient events.
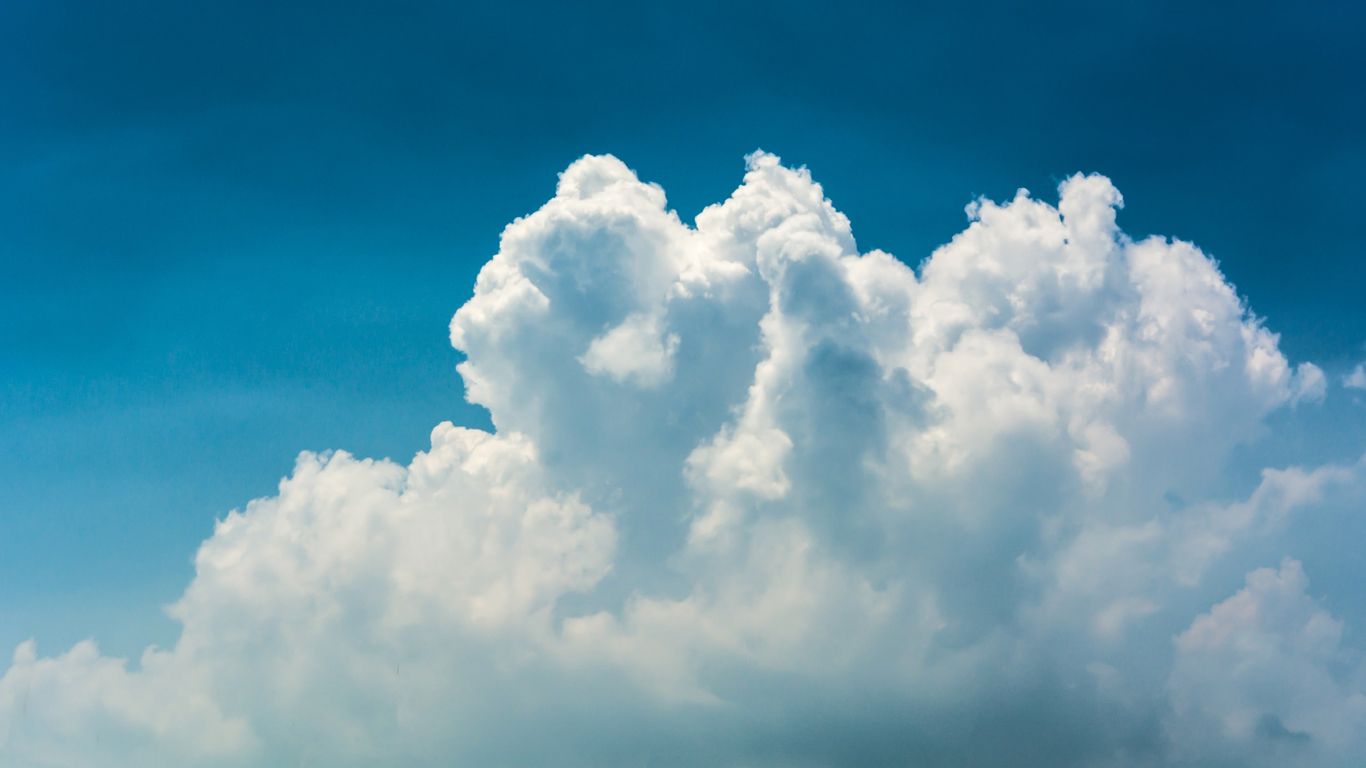
ExoGENI
ExoGENI is a national testbed created by RENCI in 2011 in collaboration with Duke University on behalf of the National Science Foundation. ExoGENI supports distributed systems and networking research using its 20 cloud sites located around the world. ExoGENI uses a unique control software framework developed by RENCI and Duke called ORCA. ExoGENI is part of the GENI federation of testbeds.
Expanding the Utility of Prenatal Genetic Testing
Expanding the Utility of Prenatal Genetic Testing focuses on developing novel approaches to identifying fetal genetic conditions in utero. The placenta and fetus shed genetic material into maternal circulation, known as cell-free fetal DNA (cffDNA). Sequencing cell-free DNA in maternal circulation, therefore, can provide information about the genetic makeup of the fetus. By both adapting and employing the latest sequencing chemistries and developing novel statistical models, we hope to better characterize fetal genetic disorders with a non-invasive approach. Diagnosing genetic disorders in utero will eventually enable better care in the immediate neonatal period and the development of in utero gene therapy and other treatments.
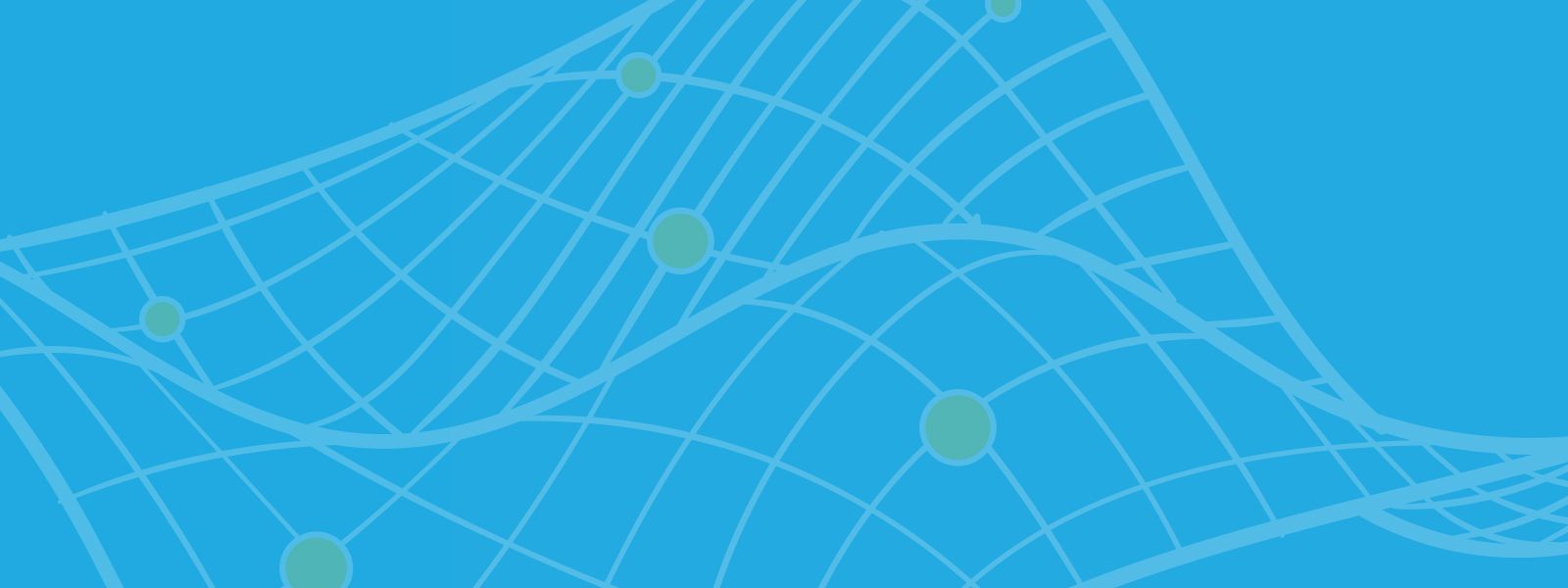
FABRIC
FABRIC is a unique national research infrastructure to enable cutting-edge and exploratory research at-scale in networking, cybersecurity, distributed computing and storage systems, machine learning, and science applications.
It is an everywhere programmable nationwide instrument comprised of novel extensible network elements equipped with large amounts of compute and storage, interconnected by high speed, dedicated optical links. It will connect a number of specialized testbeds (5G/IoT PAWR, NSF Clouds) and high-performance computing facilities to create a rich fabric for a wide variety of experimental activities.
FABRIC Across Borders (FAB)
Global science relies on robust, interconnected components - computers, storage, networks and the software that ties them together - collectively called the scientific cyberinfrastructure (CI). Improvements to individual components are made at varying paces, often creating bottlenecks in the flow of information - the scientific workflow - and slowing down scientific discovery. FABRIC Across Borders (FAB) enables domain scientists and CI experts to jointly develop a more tightly integrated, flexible, intelligent, easily programmable workflow that takes advantage of rapid changes in technology to improve global science collaboration. FAB enables domain scientists to perform global, end-to-end experimentation of new CI workflow ideas on a platform with one of a kind capabilities. The project expands the NSF-funded FABRIC testbed to encompass four additional, International locations, creating an interconnected resource on which an initial set of scientists from High Energy Physics (HEP), Astronomy, Cosmology, Weather, Urban Science and Computer Science work with cyberinfrastructure experts to conduct cyberinfrastructure experiments. In addition to domain scientists, FAB collaborates in the area of Internet freedom and maintains strong partnerships with human rights groups, which serve to expand the results beyond domain sciences.
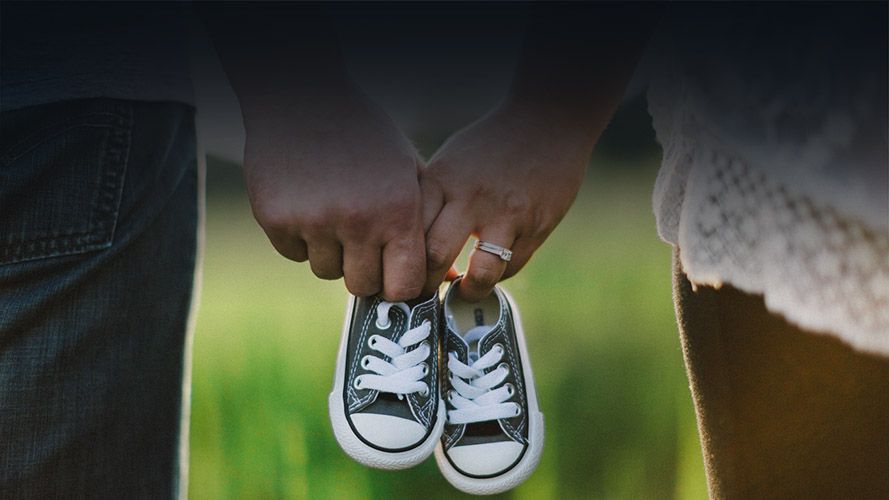
Family Planning National Training Center
The Family Planning National Training Center for Service Delivery Improvement is part of UNC's efforts on the Family Planning National Training Center (FPNTC). FPNTC, and UNC's role on it, is supported by the Federal Office of Population Affairs, under the US Department of Health and Human Services. UNC's efforts focus on growing the federally supported Title X Family Planning workforce in leading change amidst complexity and uncertainty ("adaptive leadership"). One tool we support among teams is System Support Mapping, which structures individuals' description of their role, responsibility, needs, and resource assessments in family planning initiatives. With RENCI support, the team seeks to extract insights from a set of maps to describe systems in terms of who does what, and what they need, and to support ongoing planning to support team initiatives.
FHIRCat: Enabling the Semantics of FHIR and Terminologies for Clinical and Translational Research
HL7 Fast Healthcare Interoperability Resources (FHIR) is an emerging next generation standard framework for the exchange of electronic health record (EHR) data. The FHIR specification defines a common vocabulary and mechanism for sharing and querying EHR data. All the major EHR vendors are developing standardized FHIR interfaces to their clinical data. The FHIR specification describes how FHIR data can be stored and shared using the Resource Description Framework (RDF), a standard model for data interchange on the web that has been formalized in a series of W3C Recommendations.
The FHIRCat project aims to design, develop and evaluate a novel informatics platform known as FHIRCat that will leverage Semantic Web technologies, FHIR models/profiles, and ontologies for effective standards-based data integration and distributed analytics, enabling high-quality reproducible clinical and translational research. Its goal is to build the tooling needed to enable researchers to convert FHIR data into RDF formats, validate the data using standard RDF validation tools such as ShEx, use triplestore databases to integrate FHIR data with biomedical knowledge from sources such as ontologies or databases, and to develop examples that showcase the value of these tools in better interpreting, aggregating and analyzing FHIR data.
FlyNet: An 'On-the-fly' Deeply Programmable End-to-end Network-Centric Platform for Edge-to-Core Workflows
Unmanned Aerial Vehicles (also known as drones) are becoming popular in the sky. The safe, efficient, and economic operation of such drones poses a variety of challenges that have to be addressed by the science community. This project will provide tools that will allow researchers and drone application developers to address operational drone challenges by using advanced computer and network technologies.
FlyNet will provide an architecture and tools that will enable scientists to include edge computing devices in their computational workflows. This capability is critical for low latency and ultra-low latency applications like drone video analytics and route planning for drones. The proposed work will include four major tasks. First, cutting edge network and compute infrastructure will be integrated into the overall architecture to make them available as part of scientific workflows. Second, in-network processing at the network edge and core will be made available through new programming abstractions. Third, enhanced end-to-end monitoring capabilities will be offered. Finally, the architecture will leverage the Pegasus Workflow Management System to integrate in-network and edge processing capabilities.
Providing best practices and tools that enable the use of advanced cyberinfrastructure for scientific workflows will have a broad impact on society in the long term. The project team will enable access to a rich set of resources for researchers and educators from a diverse set of institutions to further democratize research. In addition, collaboration with the NSF REU (Research Experience for Undergraduates) Site in Consumer Networking will promote participation of under-served/under-represented students in project activities.
Forecasting Coastal Impacts from Tropical Cyclones along the US East and Gulf Coasts using the ADCIRC Prediction System
Over the past two decades ADCIRC (http://adcirc.org) has become one of, if not the most widely used community modeling platform for storm surge / coastal flooding predictions across academia, governmental agencies and the private sector. The ADCIRC Prediction System (APS) manages ADCIRC on HPC resources for real-time computation of coastal hazards. This project is expanding the existing APS to incorporate new forcing mechanisms and models (NOAA’s new WaveWatch3, COAMPS-Tropical Cyclone forcing, and XBeach) and new features such as coastal erosion and sediment transport and damage assessment tools.
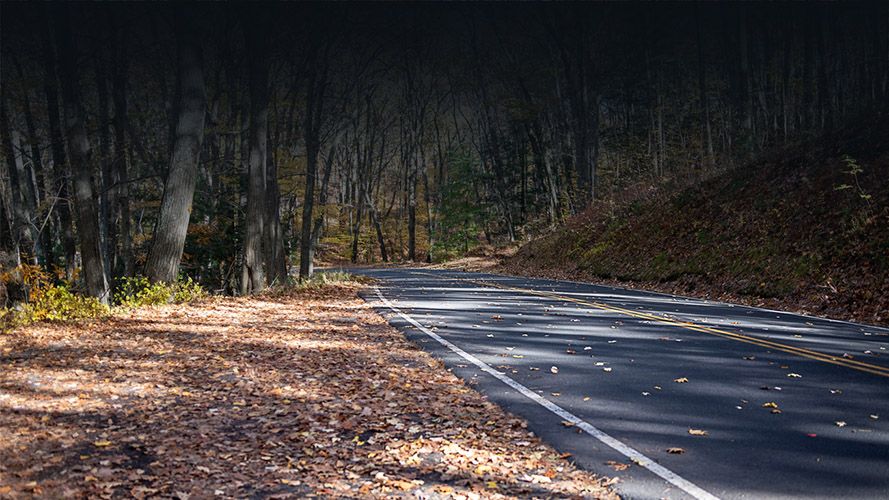
Further Development of AI Tool for Extraction of Roadside Hazards from Videolog Data and LIDAR
Severe and fatal injuries on rural roads are dispersed across many miles of roadway, leading to a labor intensive process for assessing roadside hazards. RENCI, along with the North Carolina Department of Transportation and UNC’s Highway Safety Research Center are developing an Artificial Intelligence tool to extract roadside safety-related features from NCDOT’s previously collected rural video log data.
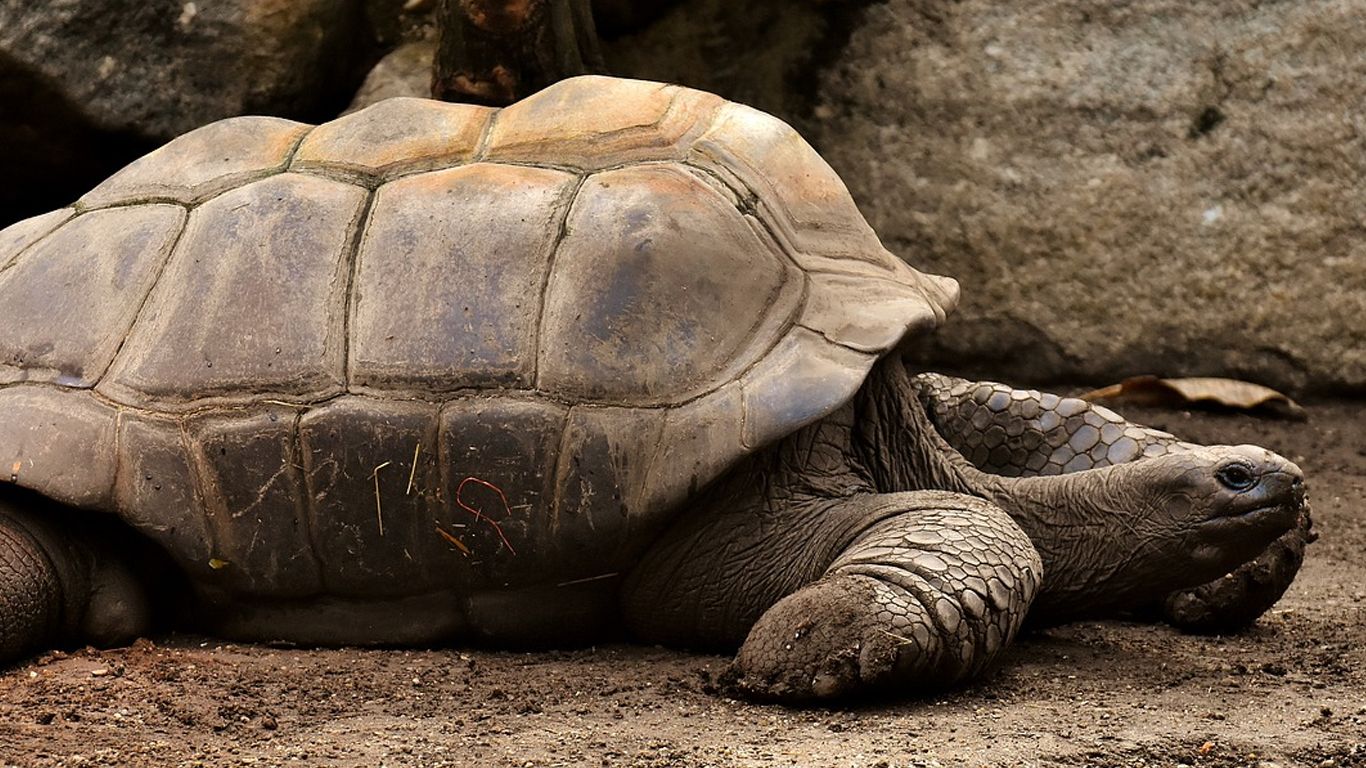
Galapagos Science Center
The Galapagos Science Center, a state-of-the-art research facility in the Galapagos Islands, was established in 2008 by the University of North Carolina at Chapel Hill and Ecuador’s Universidad San Francisco de Quito (USFQ) to promote science and education to protect the island’s unique ecosystems.
GeneScreen
GeneScreen is a screening program that aims to equip primary care clinics to detect medically actionable genetic mutations in their patients. By altering individuals and their healthcare providers to rare genetic mutations, the program can help care providers treat or even prevent diseases associated with these mutations.
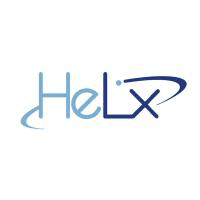
HeLx
HeLx is a command center for data science communities developed to address the need for rapid deployment of robust cyberinfrastructure in the cloud. The scalable portal allows researchers to easily ingest, move, share, analyze and archive scientific data. HeLx is designed to be flexible and customizable and empowers researchers across domains as varied as plant genomics, biomedical science, clinical informatics, and neuroscience to do work with their preferred tools close to the data in the cloud at scale.
HeLx: BRAIN-I
Through a collaboration called HeLx/BRAIN-I (pronounced brain-ee), the Stein Lab and the Neuroscience Microscopy Center uses RENCI’s HeLx framework to make high resolution microscopy images more manageable, shareable, and thereby more usable for research. The goal is to allow Stein to upload massive image files into BRAIN-I in order to view, share and analyze those images using Deep Learning methods, track and understand the origins of the data, and make the images and related analysis data discoverable to researchers at UNC and elsewhere. Use of the integrated Rule Oriented Data System (iRODS) also enables users to apply data management policies specific to the lab and the research project while keeping the data secure.
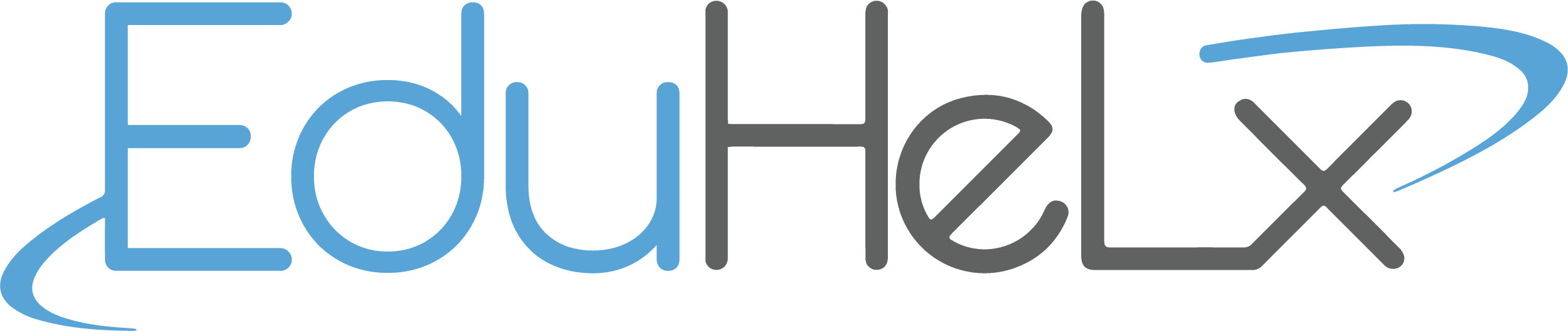
HeLx: EduHeLx
EduHeLx was born out of HeLx as an education-focused instance providing cloud-based programming. EduHeLx has been deployed in the UNC-Chapel Hill course, COMP 116: Introduction to Scientific Programming, successfully enabling students to code in the cloud. In the same ways that HeLx is designed to be a flexible and fully-customizable space which empowers researchers to easily work with their data, EduHeLx empowers students to explore the field of data science in an environment which prioritizes student investigation, focus, and cooperative discovery. EduHeLx instances are launched for each individual educator and course, making them fully customizable and unique for the students and material that will be a part of that classroom. This flexible yet powerful infrastructure creates an ideal digital learning space for students both new and experienced with data science.
HeLx: ReCCAP
The RENCI Software Architecture Group participated in a team science effort including the Gillings School of Public Health, UNC ITS, the High Throughput Sequencing Facility, TraCS, The UNC Viral Genomics Core and other groups to develop the ReCCAP analytics environment. It leverages RENCI's HeLx data science platform to support the campus effort to surveil selected UNC researchers for COVID-19 as they returned to campus in June 2020. ReCCAP provides technical interfaces to the High Throughput Sequencing Facility (HTFS), the TraCS RedCAP clinical data instance used to manage the study, and to UNC ITS services. HeLx leveraged the UNC ITS' Carolina CloudApps for the effort which ultimately allowed timely data collection and analytics of COVID-19 screenings for researchers returning to campus.
HuBMAP HIVE Collaboration Component (CC)
The HIVE Collaboration Component (CC) developed and provided robust collaboration strategies and resources in order to catalyze the Human BioMolecular Atlas Program (HuBMAP) goal of mapping the human body at high resolution to transform our understanding of tissue organization and function. The HIVE CC vision included cultivating a climate and culture that inspires collaboration, learns from the community, and is agile in the way we experiment with, adopt, and contribute to best practices. HIVE CC worked to enable successful team science with activities that enhance the collaborations and knowledge exchange among HIVE Collaboratory members, the HuBMAP Consortium, and external research communities.
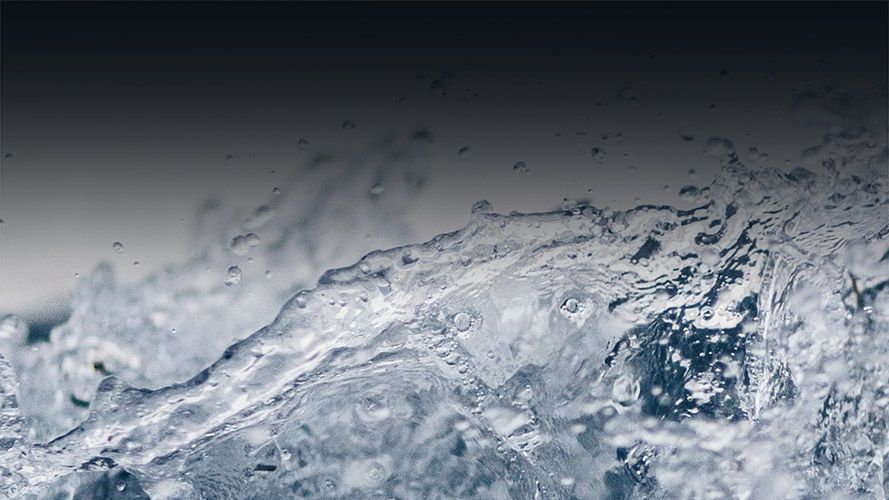
HydroShare
HydroShare is an online collaborative system to support the open sharing of hydrologic data, models, and analytical tools. It is operated by the Consortium of Universities for the Advancement of Hydrologic Science Inc. (CUAHSI) as one of its data services to advance hydrologic science by enabling individual researchers to more easily share products resulting from their research—not just the scientific publication summarizing a study, but also the data, models, and workflow scripts used to create the scientific publication and reproduce the results therein.
RENCI was a key player in the development of HydroShare. The initial HydroShare 1.0 award from 2012-2017 led to the HydroShare 2.0 award spanning 2017-2021 as well as five directly derivative awards spanning 2017-2025 and is indirectly referenced on three additional funded awards. Of significance, RENCI leads a project to generalize the HydroShare codebase which actively serves as data science cyberinfrastructure, along with PIVOT and iRODS, for the NIH Data Commons and related awards. HydroShare recently surpassed 14,000 registered users.
Imageomics Institute
The traits that characterize living organisms—in particular, their morphology, physiology, behavior and genetic make-up—enable them to cope with forces of the physical as well as the biological and social environments that impinge on them. Moreover, since function follows form, traits provide the raw material upon which natural selection operates, thus shaping evolutionary trajectories and the history of life. Interestingly, most living organisms, from microscopic microbes to charismatic megafauna, reveal themselves visually and are routinely captured in copious images taken by humans from all walks of life. The resulting massive amount of image data has the potential to further our understanding of how multifaceted traits of organisms shape the behavior of individuals, collectives, populations, and the ecological communities they live in, as well as the evolutionary trajectories of the species they comprise. Images are increasingly the currency for documenting the details of life on the planet, and yet traits of organisms, known or novel, cannot be readily extracted from them. Just like with genomic data two decades ago, our ability to collect data at the moment far outstripts our ability to extract biological insight from it. The Institute will establish a new field of IMAGEOMICS, in which biologists utilize machine learning algorithms (ML) to analyze vast stores of existing image data—especially publicly funded digital collections from national centers, field stations, museums and individual laboratories—to characterize patterns and gain novel insights on how function follows form in all areas of biology to expand our understanding of the rules of life on Earth and how it evolves.
Immune Cellular Functions Inference (ImmCellFie)
ImmCellFie is a portal for inferring cellular function given gene expression samples and their phenotypical data, based on the CellFie (Cellular Functions Inference) approach. CellFie combines a detailed systems biology input/output approach with the simplicity of enrichment analysis. The CellFie method involves generating a set of curated model-derived “metabolic tasks,” which are precomputed sets of genes that together consume a metabolite at the start of a pathway and produce a final metabolic product of interest. Using CellFie, one can overlay transcriptomic or proteomic data onto these precomputed gene modules to predict pathway usage for each metabolic task, thus providing properties-relevant interpretation of how changes in complex omics experiments modify cell or tissue metabolic function.
The ImmCellFie Portal makes CellFie accessible to the broader immunology community and beyond by providing an easy-to-use and easy-to-extend webportal housing the CellFie toolbox. ImmCellFie integrates ImmPort data with other data sources enabling users to process and compare data using the CellFie algorithm. ImmCellFie includes interactive visualization tools including heatmaps, treemaps, and Escher pathway visualizations to facilitate exploration and interpretability of CellFie results.
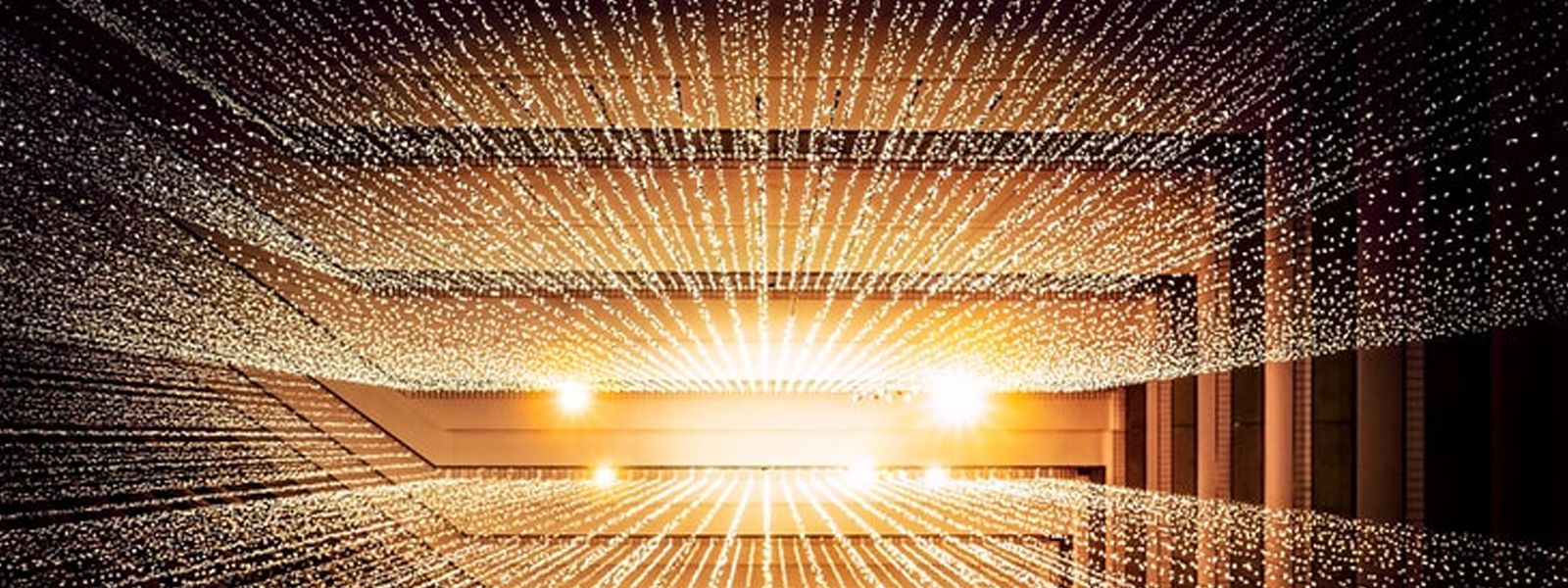
ImPACT
Scientific progress today requires multi-institutional and cross-disciplinary sharing and analysis of data. Many disciplines, such as social and health-related sciences, face a web of policies and technological constraints on data due to privacy concerns over, for example, Personal Health Information (PHI) or Personally Identifiable Information (PII). Issues of privacy, safety, competition, and ownership have led to regulations controlling data location, availability, movement, and access. Compliance poses obstacles to traditional data-processing practices and slows research; yet, increasingly, pressing scientific and societal problems demand collaborative efforts involving data from multiple stakeholders.
ImPACT (Infrastructure for Privacy-Assured CompuTations) will free researchers to focus more fully on science by supporting the analysis of multi-institutional data while satisfying relevant regulations and interests. It is designed to facilitate secure cooperative analysis, meeting a pressing need in the research community. ImPACT seeks to develop an integrative model for management of trust, deploying a collection of supportive mechanisms at scale into a model cyber-infrastructure. The project develops methodologies with best practices in networking, data management, security, and privacy preservation to fit a variety of use cases.
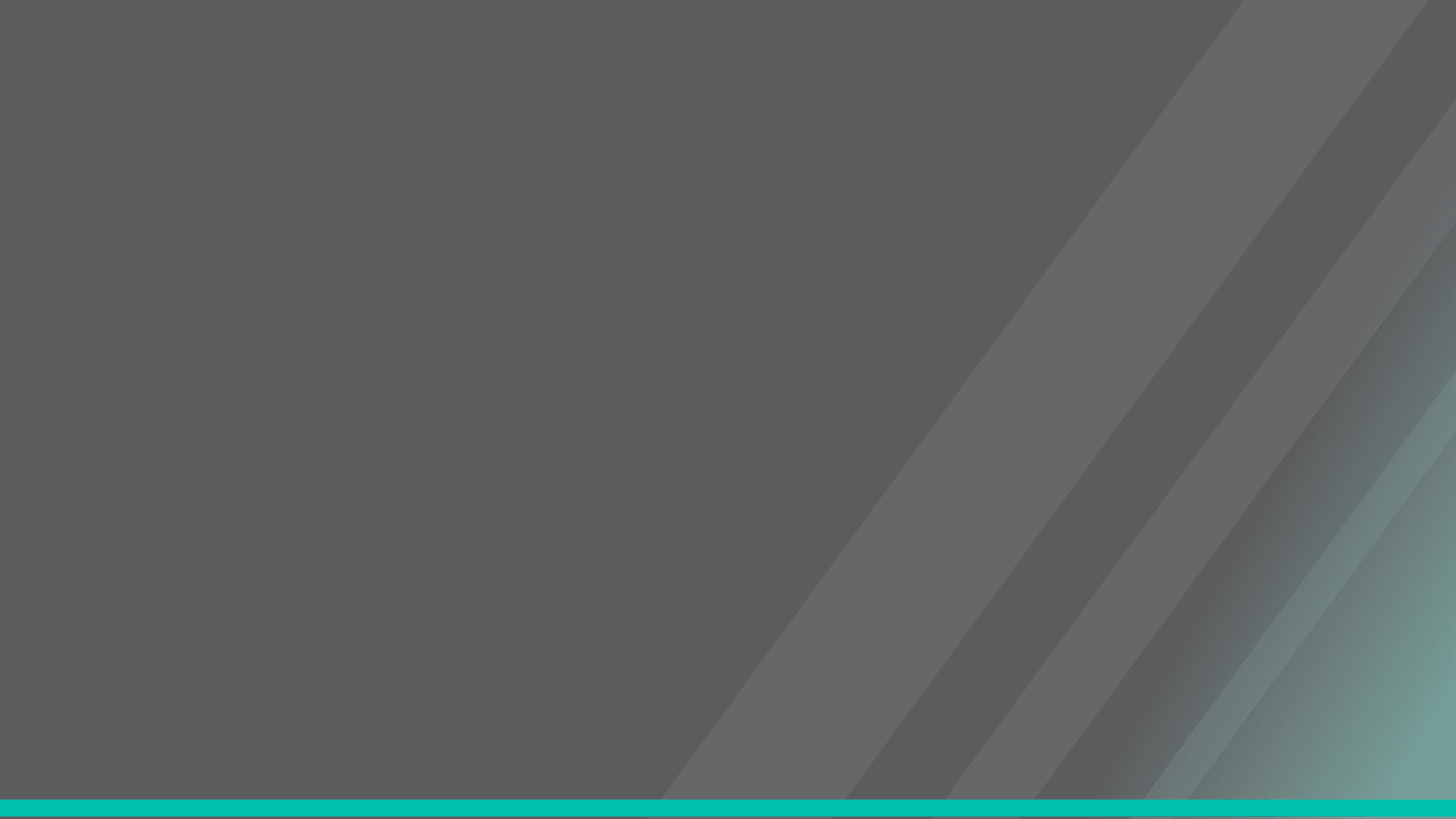
iRODS
The Integrated Rule-Oriented Data System (iRODS) is open-source data management software that enables research and commercial organizations, government agencies, and mission critical environments worldwide to test, document, control, and protect their data.
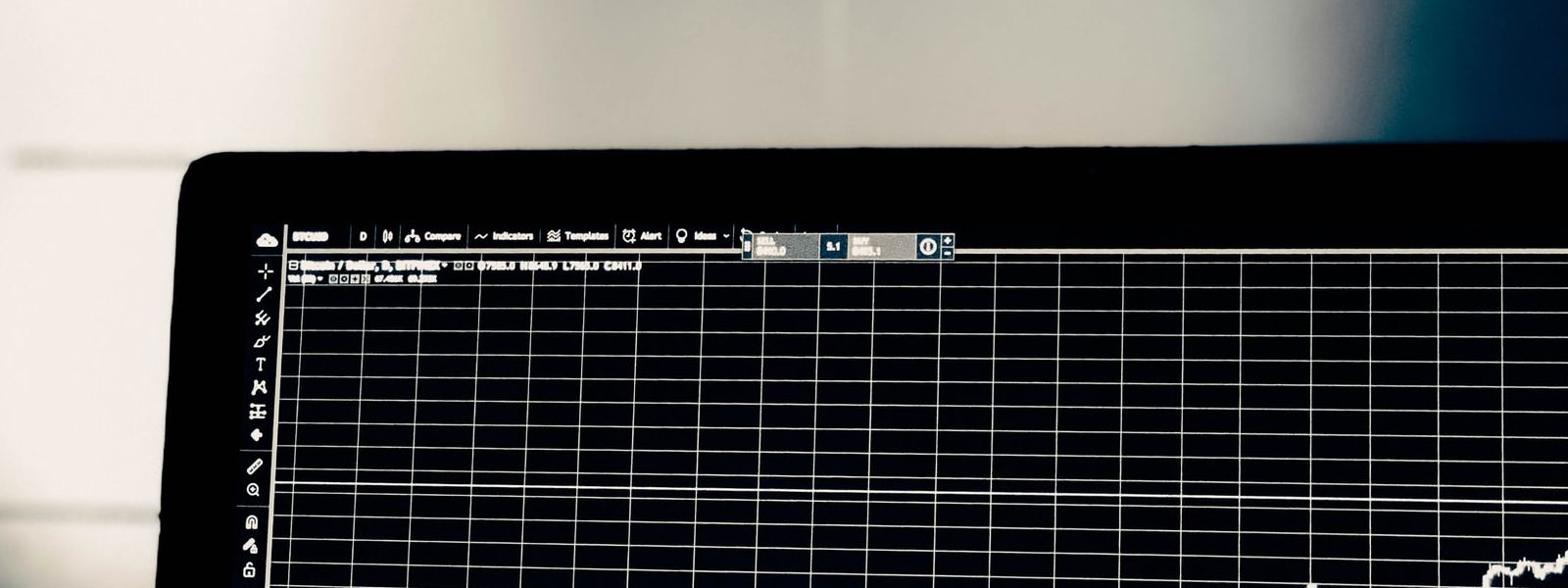
LASSaRESS
LASSaRESS aims to create a low cost, self-configurable, highly flexible, mobile system that can locate oil leaks and other contaminants with minimal human intervention.
Many Core Processors and Workflow Study for WCOSS II
RENCI has partnered with government services provider Raytheon to study the performance of computer models on new and emerging hardware. As NOAA works to update its computer hardware through the program Weather and Climate Operational Supercomputing System II (WCOSS II), Raytheon is studying how existing models for weather, river flows, and storm surge will be able to leverage new architectures such as Intel Knights Landing and Skylake processors. Because of RENCI’s expertise in high performance computing, code analysis and tuning, and Earth science applications, Raytheon sought a partnership with RENCI to extend its existing capabilities as they pursue opportunities with NOAA and beyond.
My Health Peace of Mind
The Carolinas Center (TCC) has tapped RENCI as its technology partner for a project called My Health Peace of Mind (MyHPOM), funded by the Duke Endowment. The goal of this project is to develop a system that allows individuals to develop and share advanced care plans related to future health and treatment. The MyHYPOM platform aims to encourage people to proactively manage their health, plan ahead for health challenges, and make informed choices for themselves and their loved ones before a situation becomes critical. TCC is working with RENCI to leverage its cross-disciplinary data science cyberinfrastructure (xDCI) strategic effort to create the platform. The project will also provide opportunities to study how to change behavior related to palliative care and related health issues.
National Consortium for Data Science (NCDS)
The National Consortium for Data Science (NCDS) connects diverse communities of data science experts to support a 21st century data-driven economy by:
- Building data science career pathways and creating a data-literate workforce
- Bridging the gap between data scientists in the public and private sectors
- Supporting open and democratized data
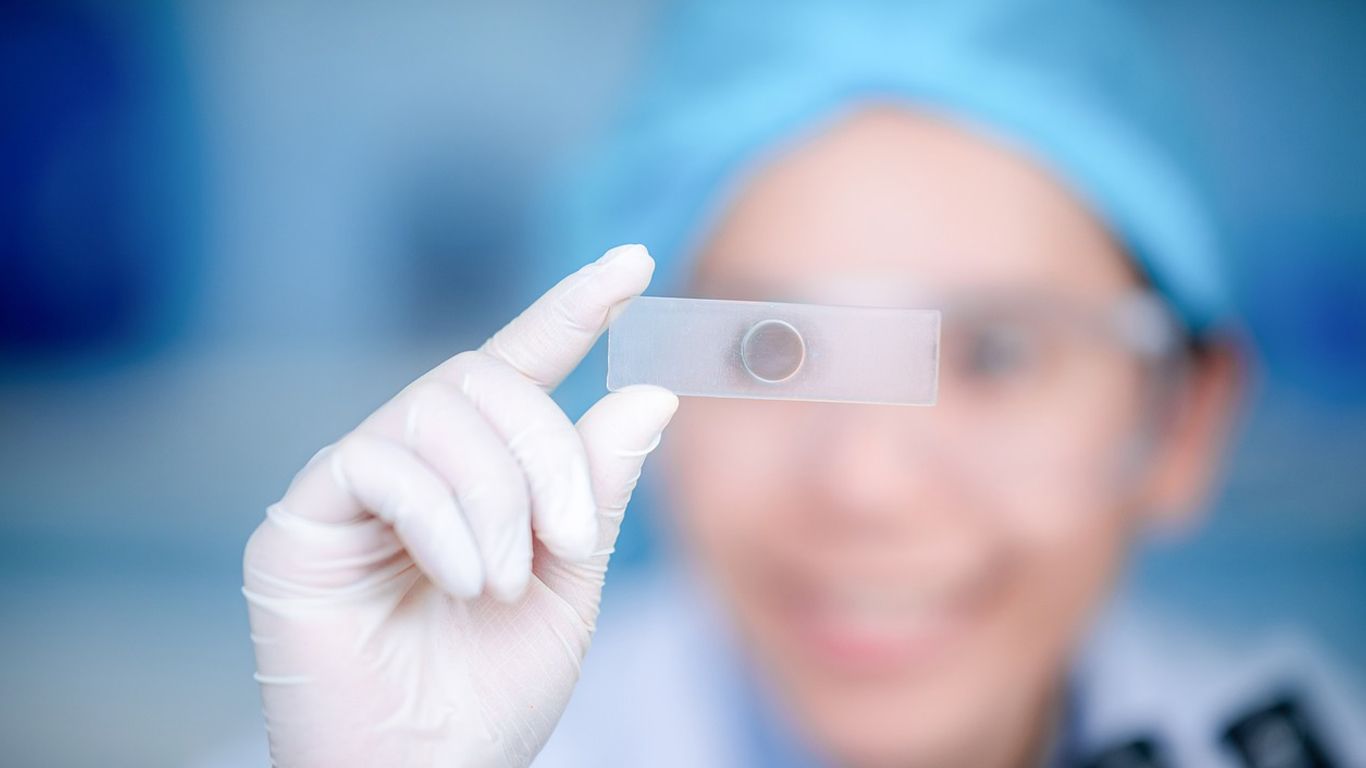
NC TraCS
The North Carolina Translational and Clinical Sciences (NC TraCS) Institute is the NCATS-funded CTSA at UNC. NC TraCS supports, funds, and connects the translational research community to improve the way biomedical research is conducted in North Carolina and across the country. RENCI leadership has served the NC TraCS Institute since 2011 as director of the Informatics and Data Science (IDSci) Component.
NCGENES
North Carolina Clinical Genomic Evaluation by Next-generation Exome Sequencing (NCGENES) is a framework for medical decision support that tackles one of the biggest challenges in genomic medicine – the need to sort through millions of genetic variants to identify the very few with actual clinical relevance.
NCNEXUS
North Carolina Newborn Exome Sequencing for Universal Screening (NCNEXUS) studied the feasibility of incorporating whole exome sequencing into routine newborn screening.
NCPI Cloud Cost Modeling
NHLBI has targeted AI image analysis as a key area for expansion in the BioData Catalyst ecosystem. However, there are currently limited to no resources available for researchers to estimate cloud costs when submitting proposals. RENCI will perform cloud cost analysis for common AI imaging applications on the BioData Catalyst powered by Seven Bridges and MIDRC platforms using publicly accessible datasets.
NeuroBridge: Connecting Big Data for Reproducible Clinical Neuroscience
Replication, mega analysis, and meta-analysis are critical to the advancement of neuroimaging research. However, these are costly and time-consuming processes, and the subjects and data are usually not similar across studies, making actual replication or meta- analysis challenging. The question is how to harness already-collected data for replication purposes efficiently and rigorously. Progress in this goal depends not only on advanced experimental and computational techniques, but on the timely availability and discoverability of the most useful datasets. Much of the present efforts on reproducibility science assumes that appropriate datasets are available. While many different neuroimaging databases exist, they have different languages, formats, and usually do not communicate with each other. Moreover, neuroimaging data are collected in hundreds of laboratories each year, forming the “long tail of science” data. Much of this data is described in journal publications but remains underutilized. A critical gap therefore exists in getting enough data of the right kind to the scientist.
NeuroBridge is a platform for data discovery to enhance the reuse of clinical neuroscience/neuroimaging data. We develop the NeuroBridge ontology, and combine machine learning with ontology-based search of both neuroimaging repositories (e.g. XNAT databases) and open-access full text journals (such as PubMed Central). The ontology leverages existing and novel ontological terms to include study types, neuroimaging description, and terms for specific clinical domains such as psychosis and addiction. We leverage technologies such as data mediation, natural language processing, text mining, machine learning, ontology look-up service (OLS), and similarity searches.
North Carolina eCrash Data Modernization Project
NCDOT/DMV engaged the University of North Carolina at Chapel Hill Highway Safety Research Center (UNC HSRC) to plan, develop, and deploy a modern web-based solution to support the law, generate accurate data that is easily accessible while simultaneously improving revenue from crash report fees. The solution would provide motor vehicle crash collection, crash-related adjudication, crash reports, data and image distribution, crash and traffic safety engineering analysis, and crash and safety prediction systems in a highly automated fashion. The crash collection effort would provide both paper and electronic submissions, but would support the goal of 100% electronic submission of crash reports. HSRC will be supported by the UNC Odum Institute, UNC Renaissance Computing Institute (RENCI), and VHB.
North Carolina Renewable Ocean Energy Program (NCROEP)
The goals of the program include:
- Advance interdisciplinary research & collaboration to bring new ocean energy technologies to the clean energy market.
- Promote testing & validation to improve efficiency, reliability, & reduce the operation and maintenance costs of ocean energy solutions.
- Inspire innovation, stewardship, and Blue Economy development through public & academic engagement.
- Lead holistic environmental assessments for the development of regulatory guidelines for responsible ocean energy advancement
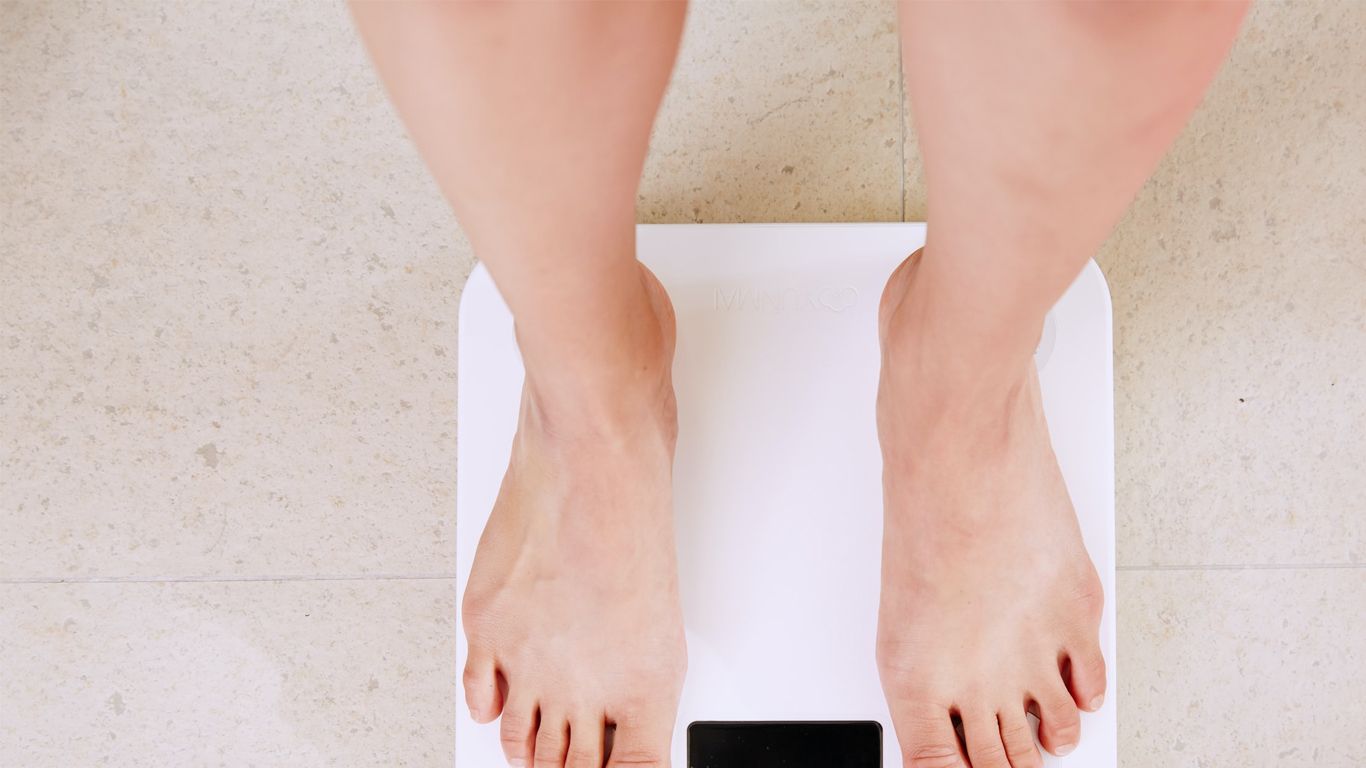
Obesity Hub
A winner of the UNC Vice Chancellor for Research’s Creativity Hubs Initiative, “Heterogeneity in Obesity: Transdisciplinary Approaches for Precision Research and Treatment” is focused on understanding why two people who consume the same diets and exercise equally can have very different susceptibility to weight gain, with the aim of developing treatment approaches that go far beyond the “one-size-fits-all” approach that is so common. The Obesity Hub is a large collaborative project with 27 faculty from 16 departments, six schools, and five centers and institutes.
Open Storage Network (OSN)
The Open Storage Network provides a cyberinfrastructure service to address specific data storage, transfer, sharing, and access challenges while enabling and enhancing data-driven research collaborations across universities and making new datasets widely available. These data sets can be used in an instructional setting to educate future researchers and data scientists. Instructors at all levels can use these data sets to expose their students to the many distinct disciplines represented on the OSN’s servers.
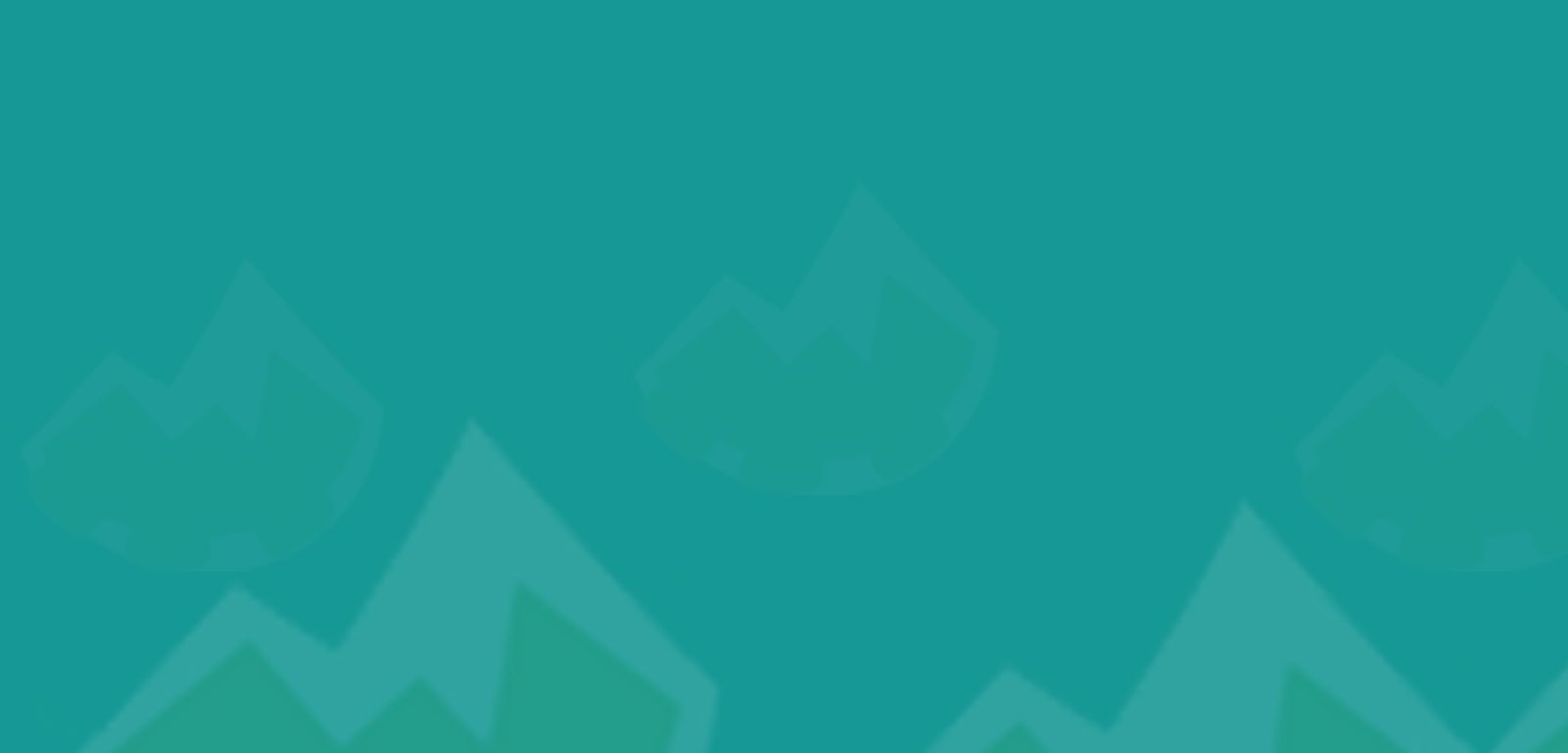
Panorama 360
The Panorama 360 project provides a resource for the collection, analysis, and sharing of performance data about end-to-end scientific workflows executing on Department of Energy facilities. In order to foster collaboration with other program participants and the broader community, the project will develop and publicize data formats and APIs to upload, download and query data. All data captured and analysis tools developed in this project are released open source and made available in GitHub.
Phyloreferencing: Creating Computable Clade Definitions in the Web Ontology Language (OWL)
Phyloreferencing aims to provide a standard mechanism for defining biological clades with precise and fully machine-processable semantics. We use ontologies and OWL (a formal ontology language developed by the W3C) to enable users to create definitions with precise, unambiguous, and fully computable semantics for any organismal clade. We call such definitions phyloreferences, in analogy to georeferences, which have enabled countless geo-enabled applications. We employ machine reasoners to determine which elements in a phylogeny match a phyloreference. We are developing the OWL ontologies, OWL data models, and tooling infrastructure needed to put our approach into practice, and to validate that it works correctly and scalably. We will also develop web applications that allow users to create, find, and reuse phyloreferences, and to apply them to phylogenies both small and Tree of Life-scale, for the purpose of integrating biodiversity data of interest. To accomplish this, we will be working with other biodiversity e-Science projects (including Open Tree of Life and the Encyclopedia of Life) as well as large organismal research collaborations.
PoSeiDon: Platform for Explainable Distributed Infrastructure
PoSeiDon aims to advance the knowledge of how simulation and machine learning (ML) methodologies can be harnessed and amplified to improve DOE’s computational and data science. PosEiDon will provide an integrated platform that helps facility operators and scientists improve the overall end-to-end science workflow by (1) predicting the performance of complex workflows; (2) detecting and classifying infrastructure and workflow anomalies and "explaining" the sources of these anomalies; and (3) suggesting performance optimizations.
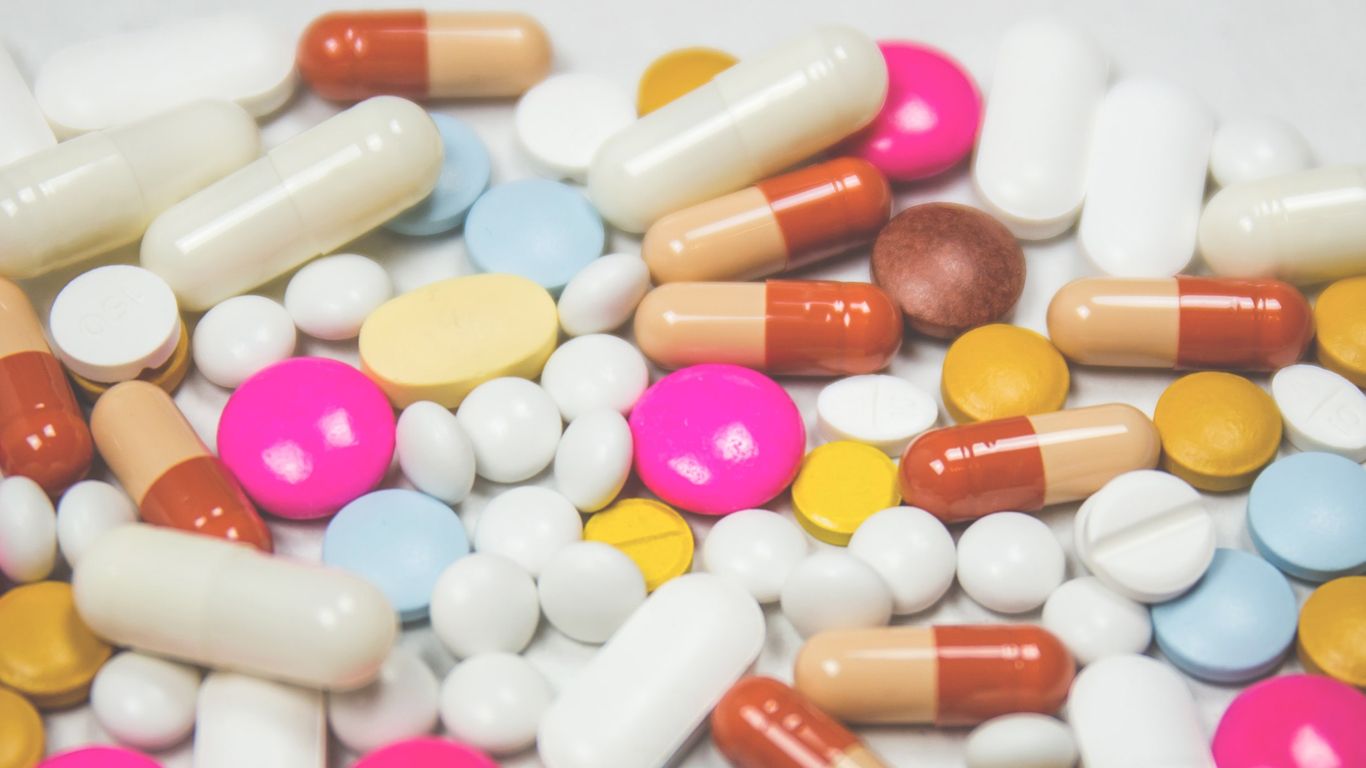
Precision Medicine and PDS
PDS (formerly PDP), developed by RENCI’s Translational Science Team, enables actionable AI integration directly with electronic health records (EHR) and computational phenotyping, while also providing an open framework and API specification for easy integration of new, third-party clinical decision support models. This design will facilitate continuous precision medicine model improvement and validation. For example, this easy-to-use framework will enable integration of new precision dosing tools to facilitate incorporation of published PK/PD/PG models into clinical workflows, as is urgently needed for provision of truly personalized medicine. PDS will also empower the clinician with tools to fight current and future SARS epidemics; these tools include guidance computed from joint modeling of patient history, disease spread, and hospital capacity.
Predictors and Mechanisms of Conversion to Psychosis
Many types of data based upon medical assays include N patients (with case/ctrl status, age of onset, survival rates, etc.) and p predictors (blood proteins, leukocytic microRNAs, clinical interview scores, genomic reads, etc.). However, conventional analyses generally fail to provide causal insight that would enable rational diagnoses or treatments. For example, genomic analyses often select ~100 predictors from many thousands using individual p-values, a hopelessly simplistic approach. RENCI has developed a completely different algorithm to identify collectively informative predictors—somewhat like choosing track team members with diverse abilities, not just the very fastest sprinters.
In our experiments with real data with N < p, N << p, and N > p, the new method appears to have superior predictive power vs. LASSO, neural nets, random forests, etc. The number of selected predictors is smaller with simpler weights and superior metrics (p-value, AUC, correlation, mean squared error). The output promotes translation of selected predictors into causality of disease, sometimes enabling backtracking to distinguish a potential trigger. Starting in 2015, four publications have applied the method to different data types in schizophrenia research. A broad paper with diverse examples, mathematical underpinnings, and open-source R and Python programs was published in Scientific Reports in 2022.
Causal network theory (e.g., Turing Prize-winning works of J Pearl et al.) is currently plagued by its prohibition of feedback loops. RENCI’s work on correlation networks (sets of blood analytes or brain regions (fMRI data) that gain or lose correlation in disease is again completely different. Selecting a threshold that approves only the strongest correlation changes identifies pairs of predictors that are unexpectedly altered. (We discard, for example, some pairs such as a pro- and anti-coagulation pair of proteins which would be expected and are observed to be almost always highly correlated.) Again, this work enables backtracking to stronger or weaker effects of common triggers, thus suggesting therapies. A paper on correlation analysis will soon be submitted to a scientific journal.
All of the above work descends from diverse psychiatric and neurological data analyses. However, far broader applications of the algorithm outside medical research are anticipated.
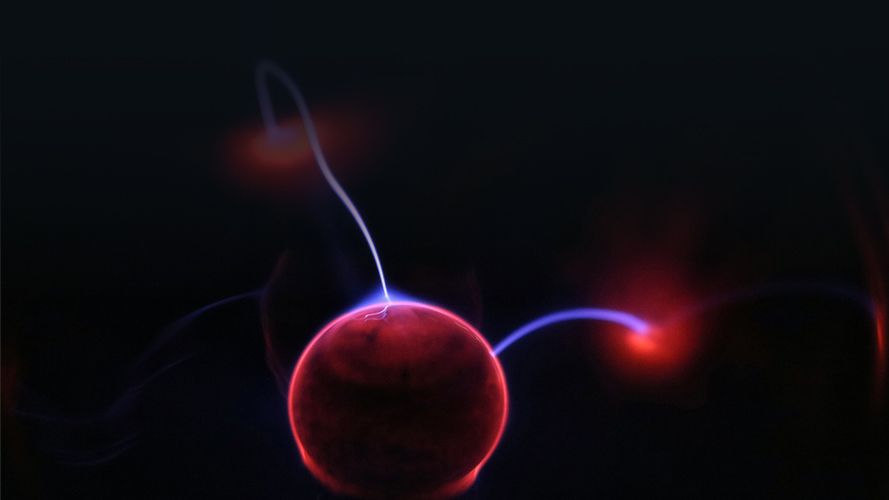
Preservice Educators Reimagining Core Experiences in Physics Teaching (PERCEPT)
PERCEPT builds upon an educational innovation—haptically-enabled science simulations (HESSs)—and seeks to extend theory to explain their impact on a persistent challenge in STEM education: elementary school teachers’ lack of comfort with and avoidance of core/fundamental physics concepts, including forces. Research has shown that elementary school teachers do not have a firm grasp of what forces are and struggle to reason about foundational physics concepts such as using the idea of forces as interactions. These ideas are "stepping stone" concepts for much of physics learning and a disciplinary core idea in the Next Generation Science Standards. PERCEPT attends to the interfaces between teaching and learning and the mediation of STEM learning through iterative use-inspired research to provide foundational knowledge for a new model of teaching and learning core physics concepts using haptic force-feedback devices combined with state-of-the-art game engine software.
Rapid Attack Detection, Isolation and Characterization Systems (RADICS)
The DoD’s Rapid Attack Detection, Isolation and Characterization Systems (RADICS) program develops innovative technologies for detecting and responding to cyberattacks on U.S. infrastructure that is essential to DoD mission effectiveness. The project focuses on early warning of impending cyberattacks, situation awareness, network isolation, and threat characterization in response to a widespread and persistent cyberattack on the power grid and its dependent systems. Technologies that could be relevant to the work include anomaly detection, planning and automated reasoning, mapping of conventional and industrial control systems networks, ad hoc network formation, analysis of industrial control systems protocols, and rapid forensic characterization of cyber threats in industrial control system devices. RENCI is part of a team led by BBN and leverages RENCI’s long-standing collaboration with the NC State FREEDM Systems Center that addresses problems related to implementing and maintaining smart power grids.
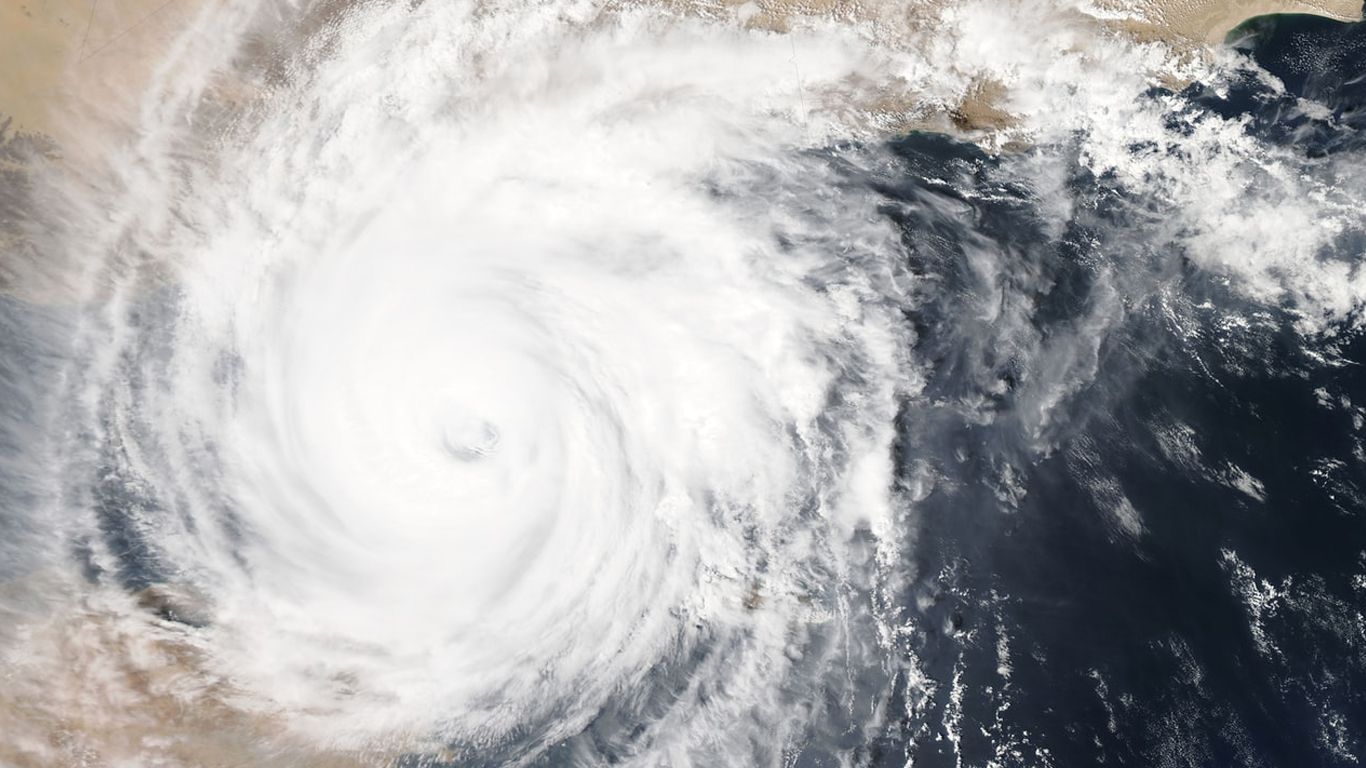
RAPID: Collaborative Research: Building Digital Infrastructure and Communities to Assess Risk of Drinking Water Hazards Caused by Hurricanes
The goal of this project is to facilitate increased access to post-hurricane water quality data through access to cyberinfrastructure, capacity development and community-building among relevant groups concerned with post-disaster water quality issues. The project featured a number of activities such as accelerating research through network-to-network collaborations, data needs and availability assessment, data collection, and educational activities focused on data curation and cyberinfrastructure.
This project grew out of prior work related to Hurricane Maria and was initiated after Hurricane Florence.
Due to the COVID-19 pandemic, the original goal to conduct two face-to-face workshops was replaced with a joint virtual three-session workshop which took place during late May and early June 2021. The content for the workshop is available in a publicly accessible GitHub repository and is available for re-use, or to make a copy to customize for additional contexts.
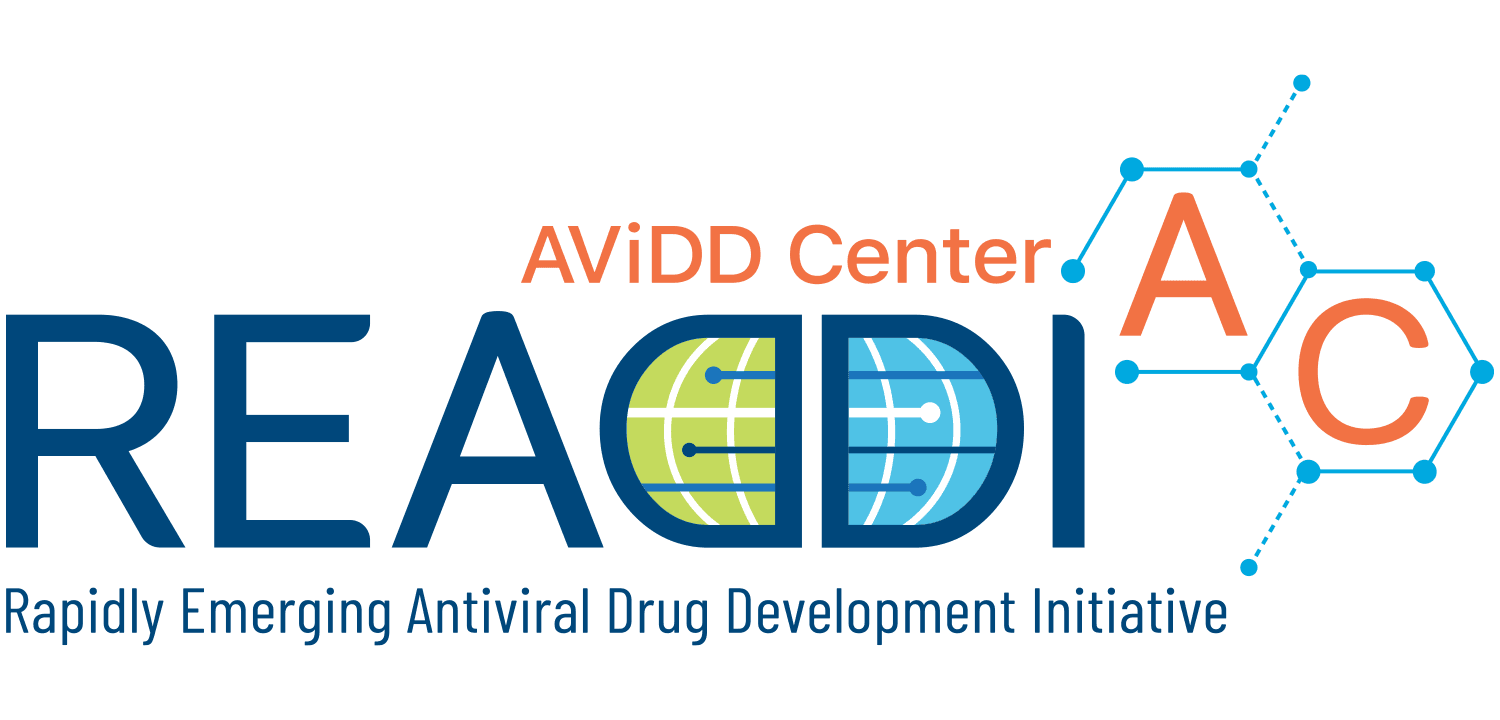
Rapidly Emerging Antiviral Drug Development Initiative-AViDD Center (READDI-AC)
Developed in response to the COVID-19 pandemic, the Rapidly Emerging Antiviral Drug Development Initiatives AViDD Center (READDI-AC) platform integrates cutting-edge discovery-based academic science with product development-oriented industrial drug development transforming the historically reactive landscape of emerging virus drug development to one that is proactive. READDI-AC components are completed in part by the creation of a FAIR-compatible data analysis platform built on HeLx, alongside Dug as a semantic search and data discovery tool.
RECOVER Data Gateway
RECOVER stands for Researching COVID to Enhance Recovery. This initiative was created by the NIH with the goal to improve our understanding of and ability to predict, treat, and prevent PASC (post- acute sequelae of SARS-CoV-2), including Long COVID. The RECOVER Data Gateway (RDG) is working with the Biodata Catalyst to provide data/metadata curation, provenance, and sustainability to include imaging, mobile health, biospecimen, and consented participant EHR data from Cohort Studies.
ROBOKOP U24
The volume of biomedical research data stored in various databases has grown immensely in recent years due to the proliferation of high-throughput biomedical ‘-omics’ technologies. Nearly all of respective databases, or ‘knowledge sources’ (KSs), address a particular area of biomedical research, leading to natural diversity but also growing disintegration between individual KSs, which generates downstream inefficiencies when mining diverse databases for knowledge discovery. Austin and colleagues described this situation as the ‘Translational Tower of Babel’ [1]. Yet, in the age of team science and cross-disciplinary research, translational research questions often require co-exploration of multiple FAIR (Findable, Accessible, Interoperable, Reusable)-compliant [2] KSs that must be connected and integrated to deconstruct the tower and identify answers and solutions.
Expanding efforts, both in academia and industry, are focused on the development of methods and tools to enable semantic integration and concurrent exploration of disparate biomedical KSs, using specially constructed biomedical ‘graph knowledgebases’ (GKBs) that support the generation of new knowledge through the application of reasoning tools and algorithms. Our team has contributed to these efforts by initiating the development of a GKB-based question-answering system termed Reasoning Over Biomedical Objects linked in Knowledge-Oriented Pathways (ROBOKOP) [3], [4]. ROBOKOP’s publicly accessible user interface (UI) [5] allows users to address both relatively simple questions such as “what genes are associated with drug-induced liver injury?” and more complex ones such as “what drugs could be used to treat airborne pollutant–induced asthma exacerbations in patients who are non-responsive to traditional medications?”
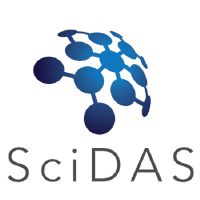
SciDAS: Scientific Data Analysis at Scale
Scientific Data Analysis at Scale (SciDAS; http://scidas.org/) researchers are developing a more fluid and flexible cyberinfrastructure for working with and analyzing large-scale data. It will combine both new and existing software to construct a system that will be efficient, practical, and user-friendly. The SciDAS system helps researchers discover data, move it smoothly across advanced networks, and improve flexibility and accessibility to national and international scientific resources. Domain scientists will be heavily involved in building the SciDAS system, providing real data sets and use cases that will help to refine the system.
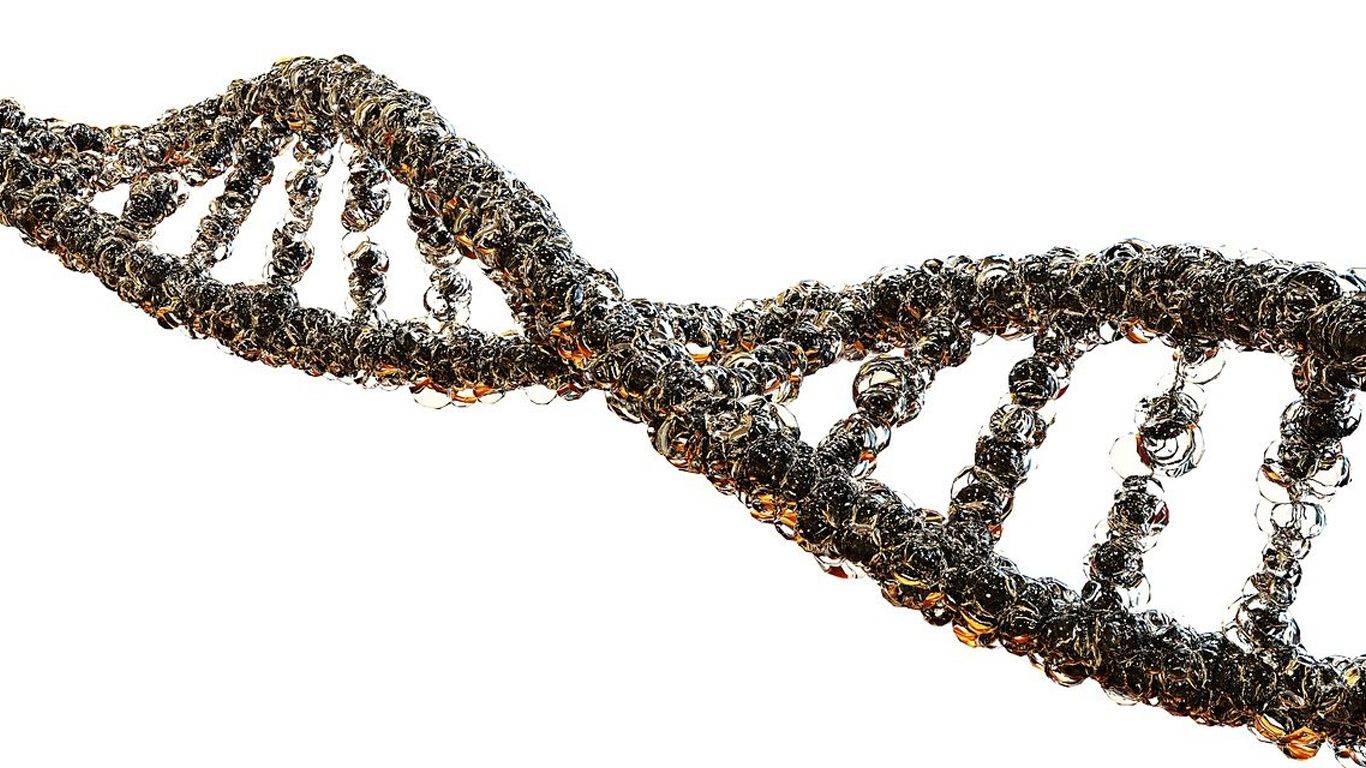
Semantic Infrastructure for Gene Ontology
The Gene Ontology (GO) project is by far the largest knowledgebase of how genes function, and has become a critical component of the computational infrastructure enabling the genomic revolution. It has become nearly indispensable in the interpretation of large-scale molecular measurements in biological research. All knowledge in GO is represented using semantic web technologies and so is amenable to computational integration and consistency checking.
Shoring up the Entrepreneurial Ecosystem through Real-Time Data Analysis
This project addressed the need for an alternative approach to firm level data collection using digital sources to inform decision making in support of entrepreneurial development. Our main objective was to provide data to entrepreneurial support agencies and organizations, enabling them to make better informed, more expedient decisions in support of innovation and firm development. These organizations would benefit not only from systematic access to real-time data sources, but equally from the ability to match and reconcile multiple data sources in support of firm level analysis. Entrepreneurial data of this nature has the potential to transform economic development analytics and guide policy towards building more resilient entrepreneurial communities.
South Big Data Hub (SBDH)
The South Big Data Regional Innovation Hub (South Hub) is one of four Big Data Regional Innovation Hubs established in 2015 by the NSF. Run jointly by Georgia Tech and RENCI, the South Hub builds public-private partnerships to address data science challenges specific to the US South.
Storm Surge and Wind Wave Simulations for Travelers Insurance
In collaboration with the Travelers Insurance Company, RENCI is conducting a suite of storm-surge simulations for three cities on the US Atlantic coast to identify specific areas that may be particularly at risk to the joint hazards of tropical cyclones and sea level rise (SLR). The three areas of interest are Charleston SC, Wilmington NC, and Virginia Beach/Norfolk, VA, where Travelers writes a substantial amount of commercial insurance. RENCI is developing the parametric hurricane descriptions and sea level rise scenarios, computing the associated storm surge response, and synthesizing the results for use in Travelers Insurance Catastrophe research group. The ADCIRC Prediction system is being used to compute the storm surge threat.
Surgical Critical Care Initiative (SC2i)
The Surgical Critical Care Initiative (SC2i) processes data from studies at world-class civilian and military research hospitals to create clinical decision-support tools that focus clinicians on the best choices for each patient.
SWIP
SWIP (Scientific Workflow Integrity with Pegasus) strengthens the cybersecurity controls in the Pegasus Workflow Management System to provide assurances with respect to the integrity of scientific data.
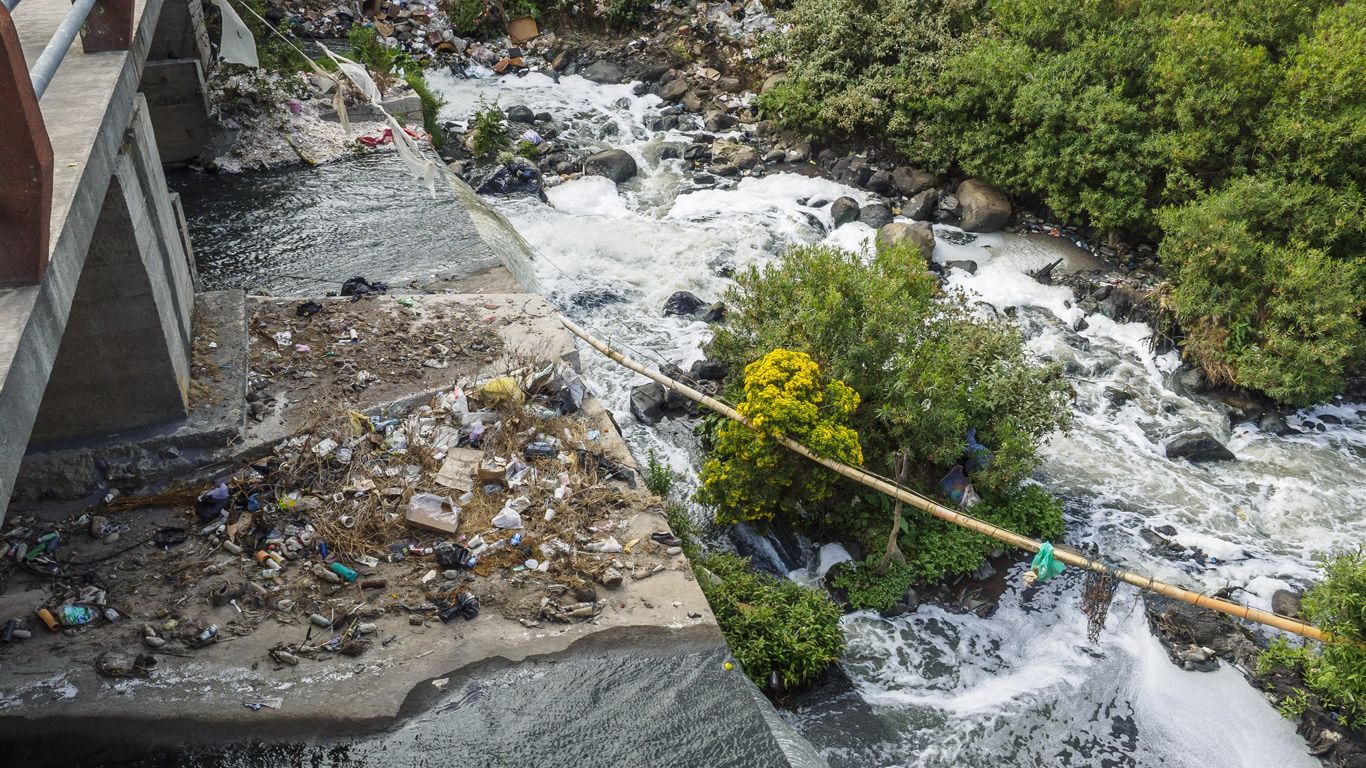
UNC Policy Collaboratory: North Carolina Per and Polyfluoroalkyl Substances Testing (PFAST) Network DataHub
RENCI co-lead the data science team for the North Carolina Per and Polyfluoroalkyl Substances Testing (PFAST) Network developing the data distribution hub for the Network. The NC PFAST Network is an effort to establish a baseline assessment of PFOA contamination of public drinking water supplies in North Carolina, to better understand the environmental and health effects of these contaminants, and to evaluate PFOA remediation technologies. The data science team is charged with developing the means to manage and make publicly available project data.
Per and polyfluoroalkyl (PFOAs) substances are chemicals with applications such as the production of non-stick surfaces and fire suppression foams. After these chemicals were found in North Carolina’s Cape Fear River, the NC General Assembly allocated funds to create a research network focused on identifying PFAS in air and water across the state, understanding its potential health impacts, and informing remediation and risk communication.
UNC Superfund Research Program - Data Management and Analysis Core (DMAC)
The interdisciplinary Superfund Research Program at the University of North Carolina at Chapel Hill (UNC-SRP) addresses serious public health challenges faced by communities in North Carolina (NC) and across the nation related to inorganic arsenic (iAs). Our unique scientific theme is “Identifying novel methods to reduce iAs exposure and elucidating mechanisms underlying iAs-induced metabolic dysfunction with a vision for disease prevention.” We address this with three aims: (1) Discover biological mechanisms and susceptibility factors underlying iAs-associated metabolic dysfunction/diabetes; (2) Develop novel methods and technologies to predict iAs contamination and reduce iAs exposure; (3) Translate the science of the UNC-SRP to key stakeholders in NC and the larger SRP program, and engage vulnerable communities. Five Cores include the Administrative Core, the Community Engagement Core, Data management and Analysis Core (DMAC), Research Experience and Training Coordination Core (RETCC), and Chemistry and Analysis Core (CAC).
RENCI’s EDS group is also collaborating on a DMAC supplemental project to develop an environmental health data visualization tool called NC Enviroscan.